Introduction
Every choice you make in your day-to-day life for your personal needs doesn’t just influence you. It leaves a mark on the environment around you. This is what we call a carbon footprint. We all have a carbon footprint, and it mostly depends on the amount of greenhouse gases we emit. These gases trap and release heat, affecting the climate negatively. As good residents of our planet, we are constantly trying to reduce our carbon footprint.
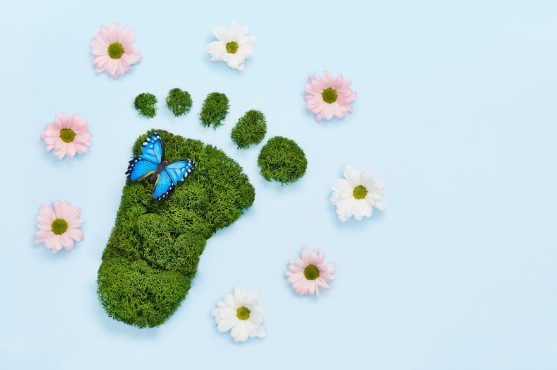
AI is a very powerful tool that can be used to combat climate change using technologies like computer vision, generative AI, IoT edge computing, and GPU acceleration. Using AI can directly result in reducing global warming and our carbon footprint.
Multiple industries are interested in lowering their emission levels as a part of their vision and goals. This has led to the global carbon footprint management market size being valued at $11 billion to date. In fact, a survey showed that 87% of CEOs with decision-making authority in AI and climate believe that advancements in AI technology are essential for reversing climate change.
In this article, we’ll explore how various AI technologies are being used to reduce carbon footprints. We’ll also discuss the related challenges and the best way to combat them. Let’s dive right in!
AI for Tracking Air Quality
If you live in a highly populated city, you must know how bad air pollution can be. We’ve reached the point where city kids may not be able to see a clear starry night. Air pollution contributes to severe health conditions, and controlling it is key to a greener future.
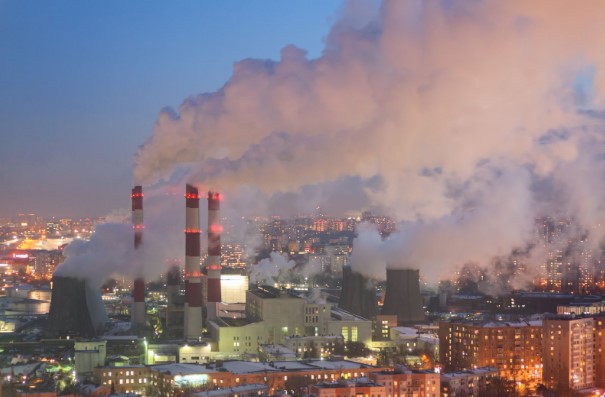
Generative AI can help authorities anticipate increases in air pollution. It can simulate various air quality scenarios based on past and current data, such as traffic patterns, industrial activities, and weather conditions. Based on these simulations, authorities can plan ahead and make decisions to reduce carbon emissions and improve public health.
Meanwhile, managing air pollution in the present requires complex numerical models that can understand weather conditions and various other factors. AI systems can easily take up this task and analyse data generated by various IoT sensors and measurements from monitoring stations to provide detailed information.
Analysis can be done in real-time with the use of edge computing in the monitoring stations. Edge computing processes data close to where it’s collected, right at the edge of the network, allowing for quicker analysis and response times. This means decisions to improve air quality can be made faster and more accurately, directly impacting efforts to reduce pollution efficiently.
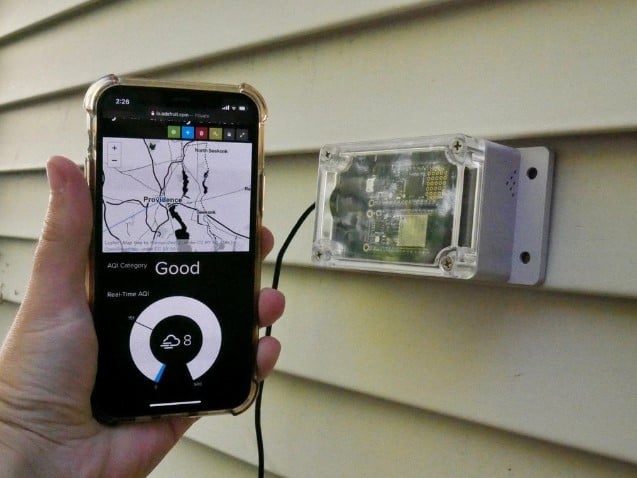
For example, let’s say a city is dealing with a lot of smog. AI can sift through data from IoT sensors throughout the city to pinpoint where the pollution is the worst. It considers things like how much traffic there is, emissions from factories, and the current weather. Based on this, the AI system might predict which neighbourhoods will have bad air quality at different times. City officials can then send out health warnings, limit cars on the road, or ask factories to cut down on work for the day. The city can get ahead of the pollution problem, keeping everyone safer and breathing easier.
Reducing Emissions in Industries Using Predictive Maintenance
In traditional maintenance, a problem occurs with a machine, and then we fix it. This method is flawed, non-reliable, and increases carbon emissions. It also lowers production as the machines won’t be able to operate during the maintenance period, which further leads to revenue loss for the company.
What if AI was able to predict problems before they occur? Fixing a problem before it occurs is much better than waiting and letting the problem get bigger. By identifying issues before they occur, companies can also better plan maintenance schedules. Better plans can reduce downtime, improve energy efficiency, and overall reduce the company’s carbon footprint.
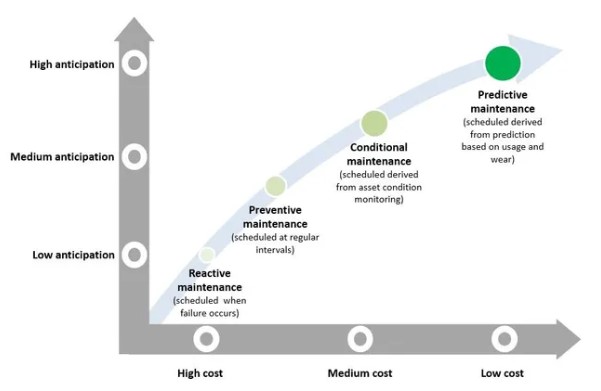
This is possible with the help of predictive analytics and generative AI and is known as predictive maintenance. Generative AI can create new unseen data by spotting trends, and predictive analytics uses historical data to help make smart choices. Combining these AI technologies, we can identify when the machine will have to undergo maintenance, giving operators time to make alternate arrangements for maintenance and ensuring stable production and revenue flow.
Here are some of the benefits of using predictive maintenance to reduce emissions:
- Less Pollution: Smoother operations mean we’re not wasting energy and pumping out unnecessary emissions.
- Reducing Costs: Catching issues early can cut down on hefty repair costs and lost production.
- Better Production: Machines that are well cared for run smoother and waste less, boosting efficiency.
- Safer Workplaces: Regular upkeep also means fewer accidents, making for a safer environment for everyone.
AI is Making Supply Chains Smarter
Supply chains are another huge factor in a company’s carbon footprint. Studies show that over 90% of an organisation’s greenhouse gases come from its supply chain. As different businesses try to reduce their carbon footprint, they are finding new and innovative ways to use AI to follow environmentally friendly practices within their supply chains. Research shows that AI will be worth $17.5 billion in the global supply chain management software market by 2028.
For instance, computer vision can be used for inventory management. Cameras are set up throughout a warehouse or storage area that continuously capture images or video footage of the products on the shelves. By analysing these images and videos, computer vision systems can monitor stock levels in real time, automatically identifying when items are running low or when there’s too much stock on the shelves. Computer vision can help businesses optimise inventory levels and reduce waste caused by overstocking or understocking.
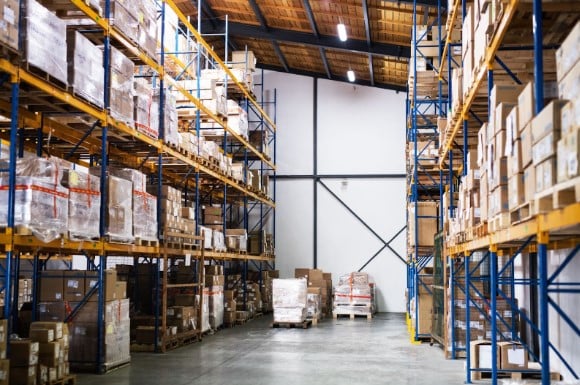
Overstocking not only ties up capital in unsold goods but also leads to waste, especially if the products are perishable. Understocking, on the other hand, can lead to missed sales opportunities and dissatisfied customers. Also, having too much inventory often means spending extra on storage and transportation, which isn’t just costly - it’s also bad for the environment due to the additional energy consumption involved.
Computer vision is just one example of AI in supply chain management. There are many different applications of AI related to reducing carbon footprints in the supply chain. GPU acceleration supercharges these AI applications, speeding up the processing of massive datasets that computer vision and other AI technologies rely on. Supply chain decisions can be made faster and more efficiently using GPU acceleration, from optimising inventory to planning eco-friendly delivery routes.
A great example of an adopter of AI in supply chain management is Walmart. They are using AI systems to plan delivery routes, which helps save fuel and reduce carbon emissions. DHL also uses a similar AI system to monitor its delivery routes and quickly spot problems like delayed packages.
Innovative AI Trends to Reduce Carbon Footprints
Lately, innovations in AI to reduce carbon footprints across various industries have been trending. Exciting applications and solutions are being worked on, and we are seeing more and more corporations take part in this trend. As more professionals take part in this movement, we are seeing climate change being controlled better and better.
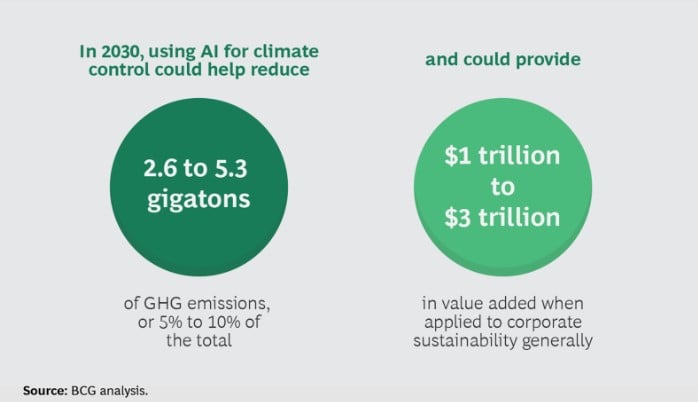
For instance, closed-loop automation systems are reshaping how operations are conducted by using real-time data to continuously monitor and adjust processes, with oil and gas companies reporting a 10-15% reduction in emissions.
AI is also making big strides with flare monitoring systems, fine-tuning how flares operate to reduce emissions by an extra 5-10%. Beyond this, AI is improving operations that run on their own, like inspections and drilling done by drones and robots. A 10-20% drop in carbon emissions can be seen by cutting down on human mistakes and the energy we don’t really need to use.
Another exciting breakthrough is digital twin technology. It builds virtual replicas of physical things and their processes. Digital twin technology lets us monitor and analyse emissions with great accuracy. It’s a big help for industries aiming to reduce their carbon footprint and meet their green goals. Digital twins are essential because they provide a clear and practical way to cut emissions down to almost nothing.
Challenges
Despite all the promising capabilities AI technologies bring to reducing carbon footprints, there are many challenges as well. One major challenge is the creation of e-waste. According to the Global E-waste Monitor 2020, the world will see an astonishing 74 million metric tons of e-waste by 2030.
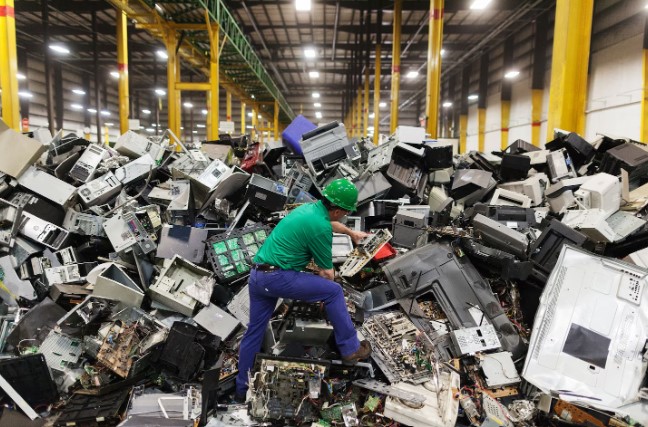
With artificial intelligence technologies being developed and integrated into daily life at a rapid pace, the world’s e-waste problem is expected to worsen as there’s a higher demand for CPUs, GPUs, and memory chips. It’s not reliable if the technology we made to reduce carbon emissions is also causing it. This problem needs to be solved as soon as possible.
Similarly, AI technologies used in data centres can have high resource utilisation, such as using lots of water for cooling. A study found that every ChatGPT conversation with 20-50 questions can use as much as a 500-millilitre bottle of water at a data centre, and in 2022, Google reported its data centres worldwide used 5.2 billion gallons of water.
Another concern is the need for more transparency in AI-related decision-making. For most people, it is unclear how machine learning models, especially deep learning networks, work and make decisions. This makes it hard to understand how AI reaches its conclusions, raising concerns about bias and accountability, especially in fields like healthcare and law. Many organisations recognise this challenge but have few comprehensive data and ethics policies in place.
Such a concern will lead to people not trusting AI. The lack of transparency and understanding of how AI makes decisions could cast a shadow over its many benefits. People need to feel confident that AI is working in their best interest, especially when it comes to critical decisions affecting health, safety, and the environment. Building this trust is essential, not just for the acceptance of AI technologies but for their successful integration into our efforts to combat climate change and protect the planet. Addressing these challenges requires expertise and care, and this is where TechnoLynx can step in to deliver verifiable and explainable AI solutions.
What We Can Offer as TechnoLynx
At TechnoLynx, we specialise in delivering custom, innovative solutions specifically tailored to your unique business challenges. We understand the difficulties of integrating AI into different industries and public sectors like transportation and power grid management. Our expertise covers enhancing AI capabilities, ensuring efficiency, managing and analysing extensive data sets, and addressing ethical considerations.
Our expertise in computer vision, generative AI, GPU acceleration, and IoT edge computing can help you explore many possibilities. We aim to push the boundaries of innovation while ensuring adherence to vigorous safety standards. For more information, feel free to contact us.
Conclusion
AI has the potential to improve various aspects of our lives, from monitoring and improving air quality to reducing carbon emissions caused by supply chains. However, integrating AI into these sectors is complex and presents many challenges.
Tackling these issues means everyone needs to work together, including AI solution providers who really get what you’re worried about and can help sort them out. At TechnoLynx, we do exactly that and are focused on pushing the boundaries of innovation while ensuring safety and transparency.
Sources for the images:
- Brent Rubell (n.d.) IoT Air Quality Sensor with Adafruit IO. Adafruit.
-DHL (2023) AI in Logistics and last-mile delivery - Envato Elements (n.d.) An interior of a warehouse.
- Envato Elements (n.d.) Steam and smoke from the chimneys and cooling towers city Central Heating and Power Plant
- Mehdi (2023) Predictive Maintenance vs Preventive Maintenance: Making the Right Choice – A Complete Guide. OCP Maintenance Solutions.
- Shehmir Javaid (2024) Sustainability & AI: 10 AI Applications in Sustainability in 2024. AIMultiple Research.
- Stephen Leahy (2017) Each U.S. Family Trashes 400 iPhones’ Worth of E-Waste a Year. National Geographic Society.