Introduction
The amount of waste we produce every day is staggering. The world generates around 2.01 billion tonnes of municipal waste every year. Every single person produces between 0.11 and 5 kilogrammes of waste every day. Thirty-three percent of that waste isn’t being handled in a safe manner, which is terrible news for the environment. Most of that waste will end up in landfills and will stay there for a very long time. That is why we need recycling.
As more people and organisations recycle, the better it is for the environment. It reduces the need for more landfills and harmful methods of waste disposal like incineration. Recycling also reduces the need for excessive extraction of natural resources. These types of extractions do more damage than good, so the less we do it, the better. Today, recycling and waste management are entering a new era, thanks to innovations in AI.
AI is a very powerful tool that can be used to improve the recycling process and waste management systems using technologies like computer vision, generative AI, IoT edge computing, GPU acceleration, natural language processing, and AR/VR/XR. A lot of enterprises are looking into incorporating AI technologies into their recycling pipelines. We can see the result of this trend by looking at the global market size of AI in recycling and waste management, which was valued at $1.98 billion in 2022 and is projected to expand and reach a value of $12.26 billion by 2030.
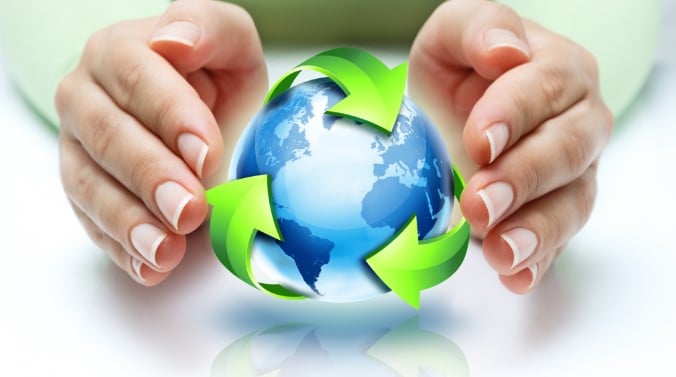
Detecting and Sorting Reusable Materials Using Computer Vision
All kinds of reusable materials show up in landfills every day. Sorting these materials is a painfully slow process. Imagine how easy it would be if a system could be made to automate the process. That’s exactly why we need computer vision. Computer vision allows systems to recognise visual inputs and use the information to take appropriate responses or actions. Much like how we use our human vision to make sense of the visual world around us, machines can derive meaningful information from digital images and videos.
A smart recycling system can combine computer vision technologies with robotics to speed up the process of detecting and sorting various types of materials. High-definition spectroscopic cameras capture images of trash moving on a conveyor belt. Computer vision and machine learning techniques are applied to the images to identify and differentiate the waste materials with incredible accuracy. The resulting data is sent as feedback to a recycling robot at the end of the conveyor belt. The robot can then take appropriate action (like sorting).
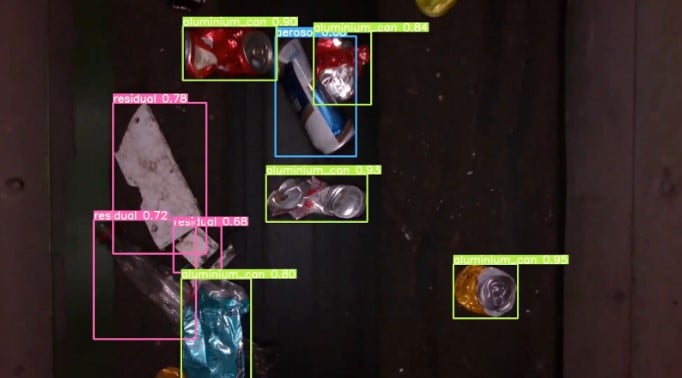
For example, Messina is becoming one of the top cities in Italy for urban waste management by using such systems at its garbage sorting and recycling facilities. Along with sorting recyclable materials, the system also gathers data about the type of garbage that is commonly collected. Government organisations and other recycling facilities can then use this data to come up with effective waste reduction strategies and public awareness campaigns.
Generative AI for Designing Reusable Materials
Most of the minerals that are extracted from the earth go into the construction and manufacturing sector. As we discussed earlier, such extractions are not good for the planet long term as they account for approximately four to seven percent of worldwide greenhouse gas emissions. To make things even worse, a lot of those extracted minerals go to waste. Over 600 million pounds of waste is produced by the manufacturing sector. We need reusable materials and new solutions to design and create such materials. Generative AI can step in as a good solution.
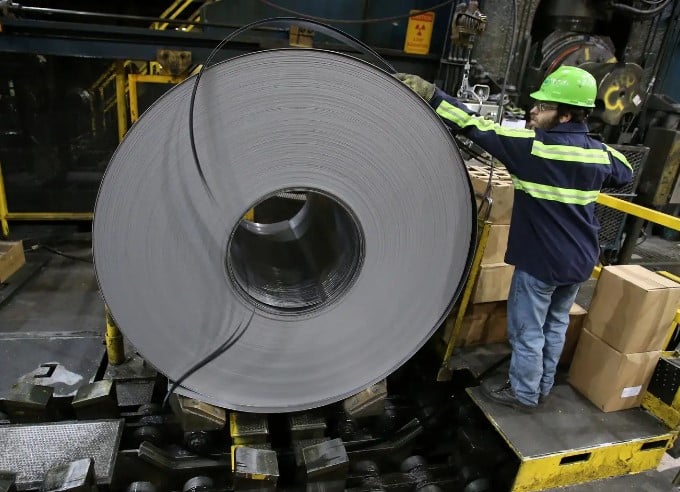
For example, generative AI can be used when recycling steel. Steel is usually recycled from scrap metal. Traditionally, recycling is done by blending this old scrap metal with large amounts of new virgin iron (which is not an ideal solution as it raises the footprint of the final recycled product). Each batch of scarp metal is different and has a unique chemical composition that lowers the strength of the new steel that will be developed. Generative AI can be used to generate custom recipes that reduce the number of additional materials added to a particular batch of molten recycled steel by learning from historical data. Such software can also help enable circular manufacturing of other materials like chemicals and cement, where manufacturers bring these materials back into the economy instead of discarding them.
Recycled Clothing Classification with IoT Edge Computing
Whenever we buy new clothes, we never really take the time to think about what happens to our old discarded clothes. In the past few decades, the production of clothes has increased five times. The annual purchase of clothes per person is about 68 clothes. In countries like South Korea, 100 billion clothes are produced every year, and almost 33 billion clothes are being discarded. Many recycling facilities are in place to solve this issue. Classifying different types of clothes is a very difficult process that requires some modern innovation. That innovation comes in the form of a combination of AI, cloud computing, and IoT edge computing. They can be used to create a clothing collection and classification system for recycling facilities.
The system can classify clothes based on image data input from IoT camera terminals installed in various clothing classification sites in various regions. The classification is done by deep learning methods using a convolution neural network (CNN). These classes can be classified as large classes and small classes. Large classes are top and bottom, adults and children, and small classes are knit, cardigan, coat, pants, skirt, etc. The results of the classification are stored in the cloud after being processed by edge devices. Edge computing allows the analysis of data captured by IoT cameras on the edge of the network before transmitting it to the cloud.
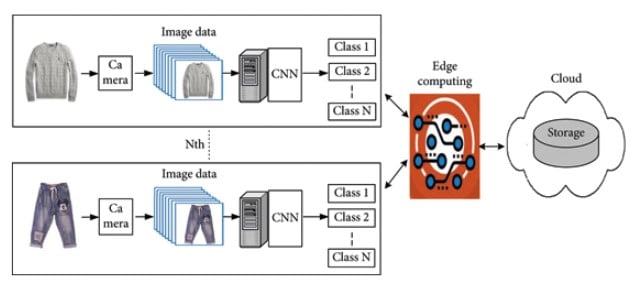
South Korea is looking into implementing such a system for classifying and recycling clothes. This system can efficiently improve the process and automation of clothing classification. It can also improve the processing of recycled clothes in various recycling facilities. It is also expected that this technology will reduce the wastage of clothing resources and improve the health of workers in such facilities.
Natural Language Processing to Extract Knowledge About Recycling
Plastic pollution has become one of the most concerning environmental issues. As the production of disposable plastic products increases, it overwhelms the world’s ability to deal with them. Most major countries are having a rough time dealing with the accumulation of plastic waste. Only less than ten percent of the seven billion tonnes of plastic waste generated globally so far has been recycled. The main problem here is the extraction of knowledge regarding recycling plastic waste. This is where AI technologies like natural language processing (NLP) step in.
The amount of literature on different approaches to managing different types of plastic waste is growing exponentially. Extracting the most relevant information from the multitude of articles, research papers, blogs, etc., is a very difficult task and a concerning issue. Such work is extremely time-consuming and effort-consuming, particularly when performed manually. NLP can be used for automatically extracting and compiling information that is most relevant to a selected category of plastics.
For example, such a system can extract research articles related to recycling different types of plastic materials. The system could use a set of keywords related to plastic recycling, such as “polyethylene recycle methods”, “polyethylene terephthalate recycle methods”, “polypropylene recycle methods”, and “polystyrene recycle methods” for relevant articles. The extracted articles are then processed to address fundamental problems related to handling plastic waste.
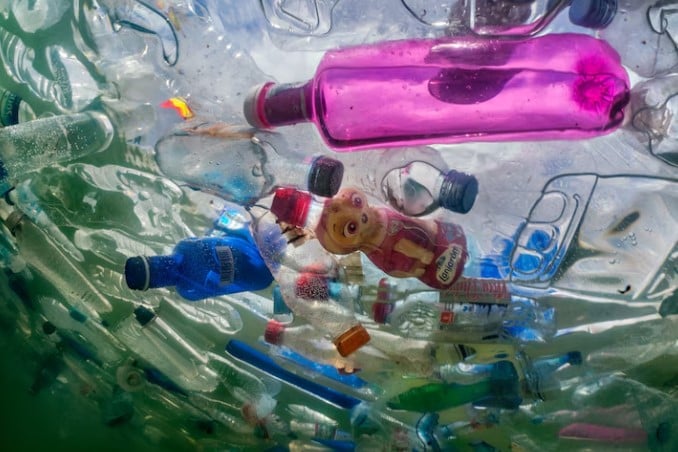
GPU Accelerated Model Training for Recycling Solutions
From model training to implementation, AI technologies struggle to work properly without the needed computational power. This is what makes Graphics Processing Units or GPUs an essential component in AI-related technologies. GPU acceleration plays a vital role in the proper functioning of recycling robots, simulations, and training large models for recycling-related applications in a real-time environment.
By partnering with GPU accelerator providers, recycling companies and organisations can create data sets and train AI models that can be integrated into recycling applications. With GPU tools and huge training datasets, recycling facilities can slash the time it takes to deploy AI models. GPU-accelerated applications can run onsite using edge computing capabilities and perform all computations locally. Models can be trained on the cloud and then deployed to edge devices at client sites. The cloud system can also process data logs and provide the client with dashboard summaries of that data.
VR Training Simulations and Games
Some of the most fascinating technologies to come out in recent years are Virtual Reality (VR), Augmented Reality (AR), and Extended Reality (XR), which is a combination of both VR and AR. VR provides a computer-generated interface that provides the user with the sensation of being entirely immersed in another environment by using realistic visuals and interactive objects. VR headsets or helmets are used to observe this environment, and the objects within it can be manipulated using smart gloves. Such AR/VR/XR technologies can be used for waste management and recycling. Let’s see how.
AI-based software solutions can be implemented along with 3D XR capabilities to create training programmes that can be used to train workers on how to handle hazardous or toxic waste like nuclear waste. The programme can be used by the expert to produce instructions for the workers to follow. They can then be trained using this technology to better handle and manage hazardous and toxic wastes without actually having to touch the real thing.
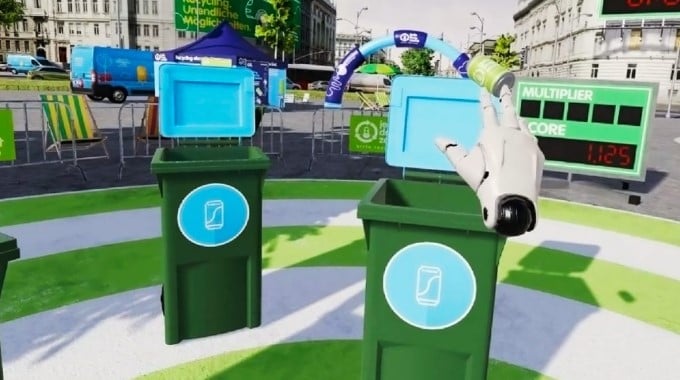
VR technology can also be used to create interactive games for children that teach them about better recycling habits. For instance, The Jede Dose Zählt campaign was a social media campaign that wanted to raise awareness about aluminium can recycling and show the audience how easy it is to recycle and do something good for the environment. They made use of a VR/AR game through which players could learn about the value of aluminium as a raw material and gain knowledge about proper waste recycling disposal systems. Fun, colourful, and set in the iconic Schwarzenbergplatz of Vienna, the objective of the game was simple, players had to throw cans into recycling containers, getting different points depending on the level of difficulty of each target.
Challenges
Despite all the promising capabilities various AI technologies bring to the recycling industry, there are some challenges as well. One major challenge is the availability of high-quality data for training AI models for recycling applications. Data on waste composition and recycling processes is often limited or of lower quality. When AI models work with small volumes of data, the model’s ability to predict accurately becomes extremely limited.
Another major challenge is regarding the complexity of different waste materials. Recycling can be done on a wide range of materials, including plastics, metals, glass, and paper, each with its own properties and recycling processes. For example, different types of metals and plastics require different recycling methods. AI systems need to be able to identify and sort these materials accurately and apply the appropriate recycling methods without any issues, which requires a deep understanding of the properties of each material.
Another concern that needs to be addressed as soon as possible is regarding infrastructure and cost. Implementing AI systems in recycling facilities needs significant investment in infrastructure and technology. This includes the cost of acquiring and installing AI systems and resources for higher computational power, as well as the cost of training staff to use and maintain the systems. For many recycling facilities, especially smaller ones, these costs can be a barrier to adopting the latest AI technology. Addressing these challenges requires expertise and care, and this is where TechnoLynx can step in.
What We Can Offer as TechnoLynx?
At TechnoLynx, we specialise in delivering custom, innovative solutions, specifically tailored to handle the challenges posed by integrating AI into various industries. Our expertise covers enhancing AI capabilities, ensuring efficiency, and managing and analysing extensive data sets.
Our expertise in computer vision, generative AI, GPU acceleration, natural language processing, AR/VR/XR, and IoT edge computing can help you explore many possibilities. We aim to push the boundaries of innovation while ensuring adherence to vigorous safety standards. For more information, feel free to contact us.
Conclusion
AI has the potential to significantly improve various aspects of recycling, from sorting out reusable materials from trash to creating interactive games to spread knowledge about the need for recycling. However, integrating AI is not simple and requires strong collaboration among all stakeholders, including an AI solution provider who knows exactly what you want. At TechnoLynx, we do exactly that and are focused on pushing the boundaries of innovation while ensuring safety and transparency.
Sources for the images:
- Acorecycling (2022) AR and VR Implementation For Recycling Habits
- Aaron Josenfczky (2023) AI is now writing “green” recipes for recycled steel
- Amit R (2023) Teaching Kids About The Significance of Recycling to Save The Planet
- Cbinsights (n.d.) Recycleye’s Product Videos.
- Randy Olson (n.d.) We Made Plastic. We Depend on It. Now We’re Drowning in It. National Geography
- Sun-Kuh Noh (2022) Deep Learning System for Recycled Clothing Classification Linked to Cloud and Edge Computing
References:
- Acorecycling. (2022). AR and VR Implementation For Recycling Habits.
- Acspublications. (2023). Recycle-BERT: Extracting Knowledge about Plastic Waste Recycling by Natural Language Processing.
- Noh, S. 2022. Deep Learning System for Recycled Clothing Classification Linked to Cloud and Edge Computing.
- Peters, A. 2023. From cheese to steel: 6 unconventional ways that AI is being used to help the planet.
- Recycleye. (2021). NVIDIA Blog: Recycleye’s AI-Driven Detection System Primed to Take a Bite Out of Global Waste.
- Recycleye. (n.d.). Messinaservizi innovates with Recycleye robot.
- Replanet. (2024). Messinaservizi innovates with Recycleye robot.
- The World Bank. (n.d.). Trends in Solid Waste Management.
- Unep. (n.d.). Our planet is choking on plastic.
- Velichko, Y. (2022). How to Make Waste Management and Recycling Smarter with Computer Vision.
- Vrisch. (n.d.. VR-Sustainability Game “Every Can Counts”.