Introduction
Our world relies heavily on energy, mostly from fossil fuels (coal, oil, and gas), which pose supply limitations and environmental challenges. Increasing energy demand from growing populations and economies stresses existing energy systems, urging a transition to sustainable sources like solar and wind. The IEA forecasts a global population of 9.7 billion by 2050 with a 25% increase in energy demand. Amidst these daunting statistics lies a beacon of hope: AI solutions for reducing carbon footprint that unlock opportunities in the energy sector.
McKinsey states that AI for energy efficiency has the potential to reduce global energy consumption by up to 10% by 2040, equivalent to approximately $1.2 trillion in energy savings. In this context of the urgent need for a transition towards sustainable energy, the role of AI emerges as a crucial enabler. This article explores the intersection of AI, energy, and sustainability, delving into the myriad ways in which AI technologies are reshaping the energy sector.
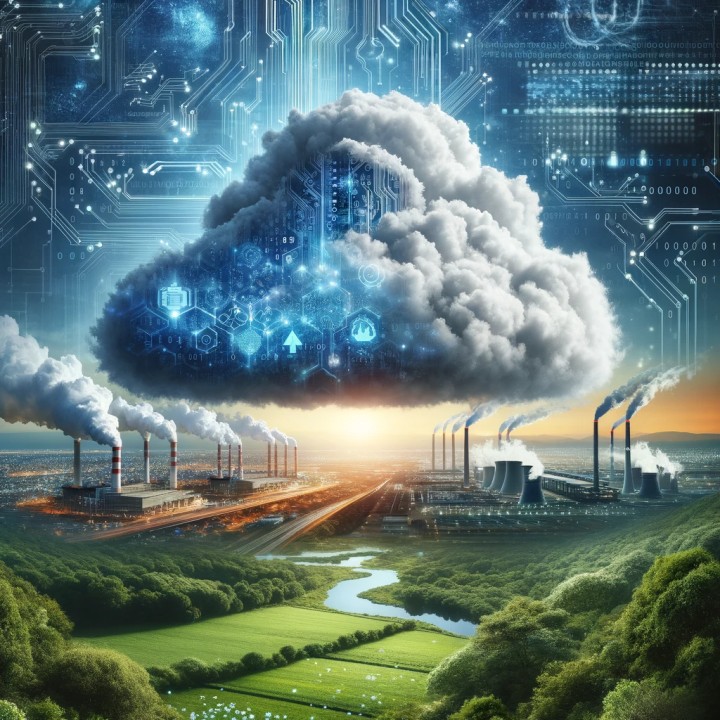
Generation Forecasting
For renewable energy, correctly forecasting power generation becomes critical in the era of renewable energy. It helps us use resources wisely and keeps our energy systems stable. AI in renewable energy offers advanced solutions like machine learning and computer vision which offers irreplaceable competences in forecasting power plants operation with the best possible precision.
AI Predictive Models for Renewable Energy
Consider, if we were able to predict future deployment trends of renewable energy sources such as solar and wind prior to their installation. AI uses complex algorithms to analyse past data, weather patterns, and other factors to forecast how much energy renewable sources will produce. This enables energy corporations and grid operators better plan for when and where to use AI in renewable energy making our electricity systems more efficient and reliable.
To illustrate, for CAISO the major source of independent power is renewable energy such as solar & wind power. AI-powered predictions made it possible for CAISO to anticipate fluctuations in renewable energy output more accurately, thus letting grid operators adjust dispatch schedules in real time.
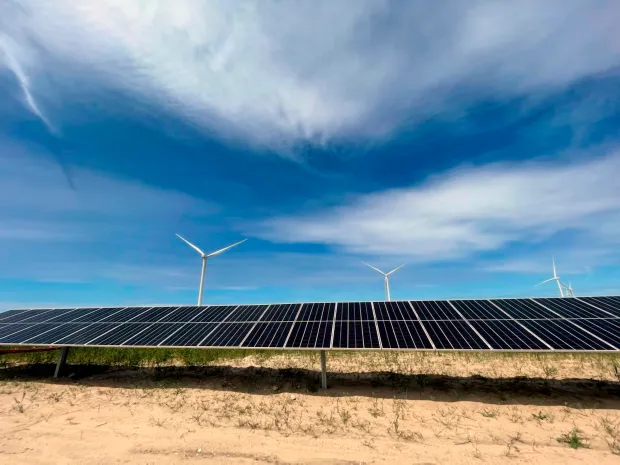
Real-time Monitoring of Solar Panel Efficiency
The effectiveness of solar panels is vital for the level of energy they can generate. But sometimes, things like dust, shade, or degradation over time can adversely impact solar panel performance. Traditional monitoring techniques often rely on periodic inspections, which may overlook real-time fluctuations in panel efficiency. That’s where artificial intelligence in solar energy comes in.
With AI-driven computer vision technology, you can watch live video feeds of solar panels and spot any issues right away. Computer Vision can detect issues like dirt, damage, or if a panel isn’t lined up properly. This keeps solar panels plants running smoothly and efficiently, giving us more clean energy for our homes and businesses.
SolarEdge integrated high-resolution cameras, analysing live video feeds of thousands of solar panels in Nevada, USA. Computer vision detects dust accumulation, shading from nearby structures or vegetation and physical damage. As a result, SolarEdge addressed issues promptly and reduced maintenance costs by 20% by prioritising interventions based on real-time data insights. It also observed a 15% improvement in energy output from its solar farms in Nevada by detecting and rectifying issues such as dust accumulation and shading.
Generating Synthetic Data to Enhance Forecasting
The problem with renewable energy forecasting is data scarcity, especially in regions with a narrow historical database and diverse environmental conditions. Through Generative AI, you can synthesise realistic data samples based on existing datasets, thereby augmenting the training data for AI prediction models. This, in turn, enhances the precision of the outcomes, particularly in those situations where the real data could be limited or incomplete.
The National Renewable Energy Laboratory (NREL) struggled to forecast wind generation in areas with limited historical data. As a result, researchers have applied AI applications in wind energy, such as generative adversarial networks (GANs) - a type of Generative AI that is trained using wind speed data from nearby regions with similar environmental conditions to generate realistic synthetic wind speed samples for the target region.
NREL’s deployment of GANs for generating synthetic wind speed data in remote coastal areas improved the forecasting accuracy by 30%, enabling more reliable wind energy integration into the local grid. This improvement in accuracy translated into more reliable forecasts for energy operators and grid managers for better resource allocation and grid balancing strategies.
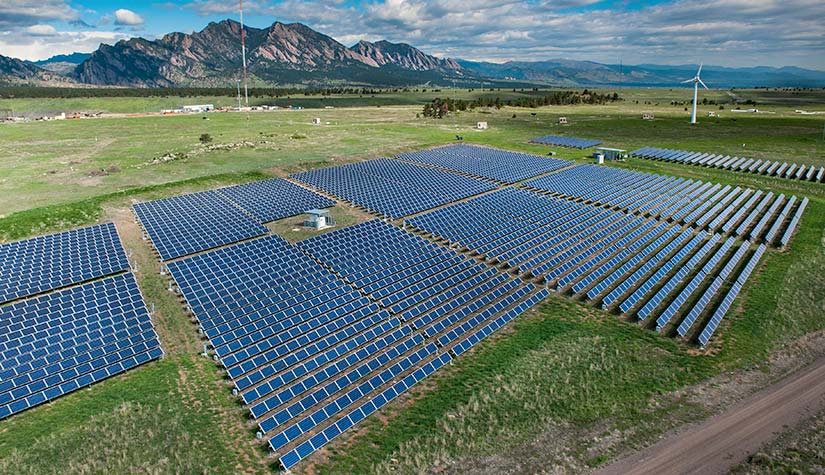
GPU Acceleration for Rapid Processing of Data
The energy sector should process a huge amount of data in real time to make timely decisions. With GPU acceleration, AI systems can process vast amounts of data in a fraction of the time, helping energy companies analyse information more efficiently and make faster, more informed decisions. GPUs (Graphics Processing Units) are specialised processors that can handle complex calculations and data processing tasks much faster than traditional CPUs.
Using NVIDIA Tesla GPUs, the National Energy Research Scientific Computing Center (NERSC) was able to reduce the time it takes to create a renewable energy data set from days to just minutes, significantly speeding up the decision-making process for grid balancing and energy dispatch. These datasets use machine learning algorithms for predicting energy demand, optimising grid operations, and forecasting renewable energy generation.
Power Plant Monitoring
Power plants are crucial for supplying energy to meet the demands of modern society. However, keeping tabs on power plants is crucial. But how do we do this efficiently?
With AI algorithms, power plant monitoring becomes a more proactive, data-driven approach to operations and maintenance. Let’s break this down into three simple steps:
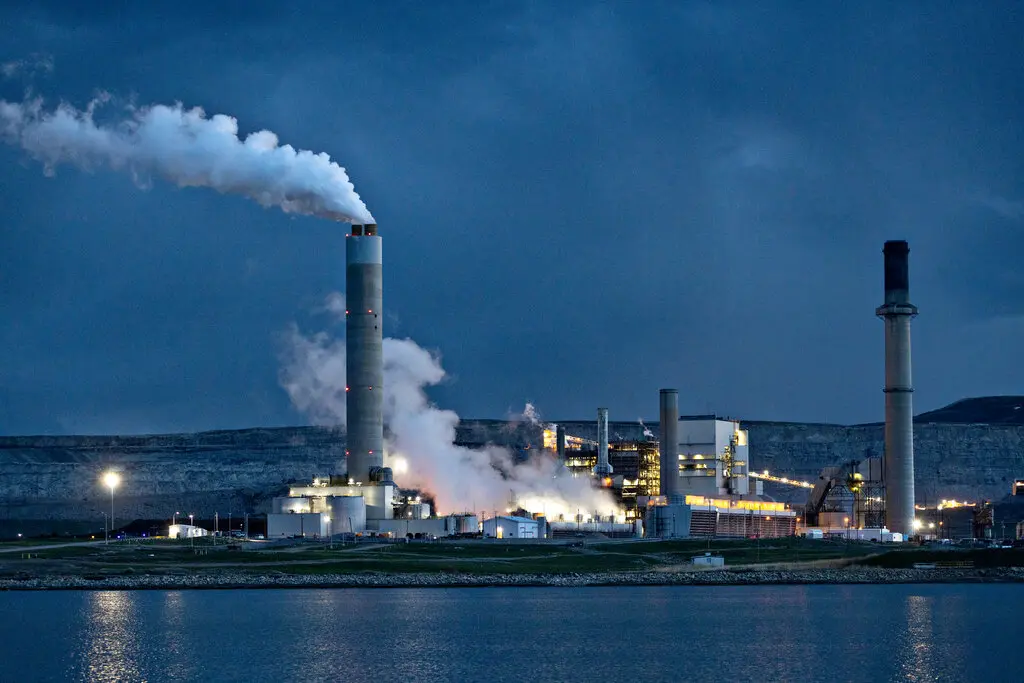
Continuous Monitoring with IoT Edge Computing
Keeping power plants running smoothly is crucial for a steady electricity supply to our homes and businesses. Traditional methods of power plant monitoring involve periodic manual inspections and data collection, which can be time-consuming and prone to human error. With IoT edge computing, power plants can monitor their operations in real-time from sensors spread throughout the plant, constantly measuring things like temperature, pressure, and vibration.
These sensors send data to a computer system and screen out abnormalities, predict failures, and optimise plant operation. Machine learning algorithms are capable of spotting the signs of instrument failure or the condition when the equipment is not operating to its full potential, allowing operators to take preventive steps to avoid downtime and improve energy efficiency.
At a thermal power plant in Ontario, Canada, implementing IoT sensors and AI algorithms for continuous monitoring led to a 25% reduction in unplanned downtime and a 10% improvement in overall operational efficiency within the first year.
Analysing Maintenance Reports and Identifying Potential Issues
Suppose you’re in charge of a power plant, and every day, your team writes reports about the power plant’s equipment, which they’ve checked and fixed. However, manually analysing these reports to identify trends, recurring issues, and emerging maintenance needs can be labour-intensive. With NLP, AI systems can automatically analyse maintenance reports, extract key information, identify patterns, and flag potential issues for further investigation.
So, instead of spending hours poring over paperwork, you can let NLP spot patterns and trends, like if a certain machine keeps causing trouble or if there’s a part of the plant that needs extra attention. So you can focus on fixing things before they become big problems. For instance, NLP algorithms can organise the maintenance events based on the equipment type, location, and severity, which allows maintenance activities to be prioritised and resources to be allocated accordingly.
Ontario Power Generation (OPG), a huge contributor to electric power in Canada, is the operator of hydroelectric, nuclear, and thermal power stations in various regions of the country. They faced challenges in managing maintenance activities and identifying emerging requirements across Canada. By integrating NLP algorithms, OPG automated the analysis of maintenance reports, extracting key information and associated locations. The NLP algorithms were trained to spot the patterns and trends in the reports, highlighting the ones that repeat frequently, indicate a developing maintenance issue, or are so crucial that the defective component must be replaced immediately.
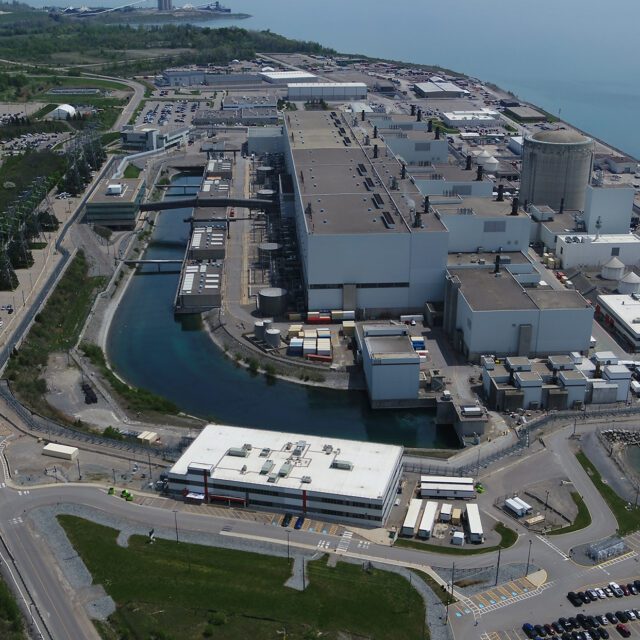
Remote Troubleshooting & Maintenance Training
Suppose you work in a power plant, where fixing machines and doing maintenance can be really tricky, especially if you’re in a remote or dangerous area. That’s where XR comes in, which lets you create immersive worlds where you can troubleshoot problems and learn how to fix things without actually being there in person. With AR glasses, you can see interactive guides and manuals right in front of you, showing you exactly what to do step by step and support from experts located elsewhere.
For example, GE Power developed an AR application for maintenance technicians to overlay digital information like equipment schematics, diagnostic data and repair instructions onto physical equipment in the power plant environment. Using a smartphone or tablet equipped with the AR application, technicians can visualise equipment configurations, identify components, and access relevant information without needing physical manuals or diagrams.
With VR headsets, GE provides technicians with a virtual environment that replicates the layout and operation of various power plant systems. With interactive scenarios, technicians can practise troubleshooting common equipment faults and performing maintenance tasks in a safe and controlled setting. Also, GE Power uses XR technology for real-time collaboration between on-site technicians and remote experts. Remote experts can view the technician’s field of view in real-time, annotate images, and provide step-by-step guidance to facilitate troubleshooting and repair activities.
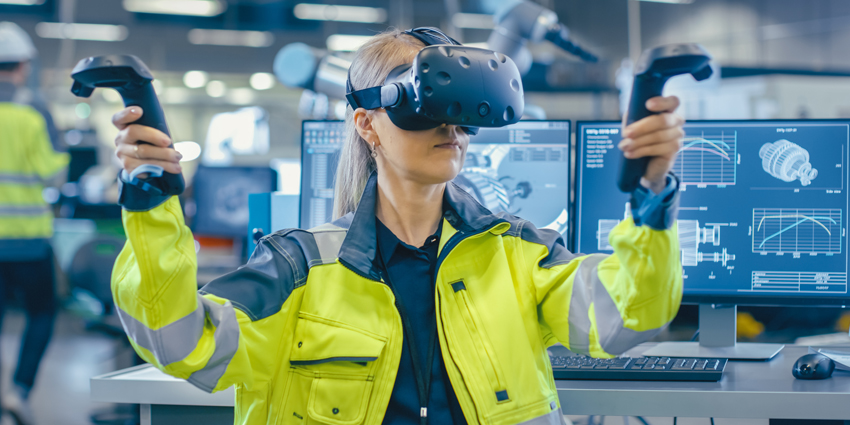
Gas and Oil Exploration
It’s the process of extracting the important energy sources, essential for keeping our lights on and our cars running. Sometimes, the clues about where gas and oil might be hiding are really hard to see. Also, drilling in the wrong place can be really expensive and bad for the environment. That’s where artificial intelligence, or AI, comes in to save the day! By using AI, we can choose drilling locations that have less impact on nature and wildlife. This helps us protect our environment while still meeting our energy needs. This means it costs less money to find gas and oil, making energy more affordable for everyone.
Applying Computer Vision for Analysing Seismic Data
Ever wondered how oil and gas research teams discover where oil and gas can be hiding deep underground? The information derived from seismic data - acquired via imageries - gives vital details about geological formations that may contain gas and oil. Nevertheless, the laborious work of the seismic experts might cause some errors. Combining computer vision algorithms to seismic data with AI system’s ability to interpret complex seismic images, identify potential hydrocarbon reservoirs and characterise subsurface features with unprecedented accuracy.
These algorithms apply pattern recognition & image processing techniques to detect subtle seismic anomalies indicating oil and gas accumulations, helping the exploration teams to recognize the promising drilling locations accurately. For instance, Chevron collected all seismic data in their Gulf of Mexico projects and manually analysing it required significant time and resources. By automating the analysis of seismic data using computer vision, Chevron’s exploration teams were able to rapidly identify promising drilling locations while minimising the cost of drilling dry wells.
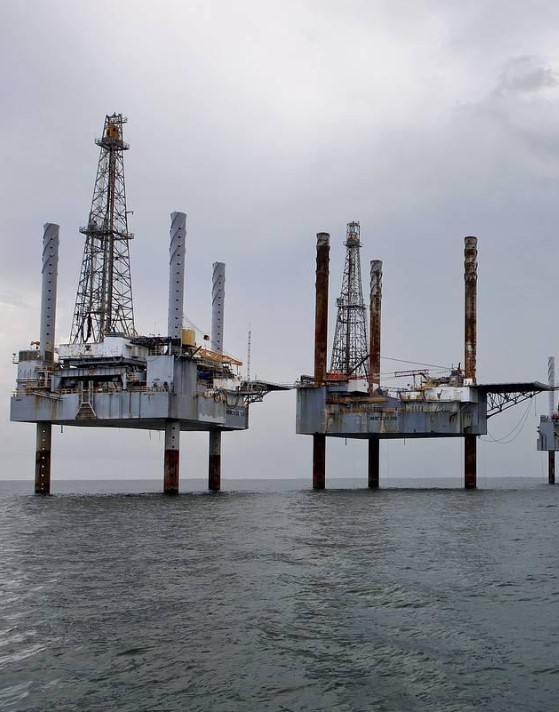
Predicting Resource Locations & Complex Geological Data
With the integration of generative AI algorithms like Generative Adversarial Networks (GANs) and Variational Autoencoders (VAEs), companies can simulate geological formations and predict resource locations with unprecedented accuracy. Generative AI models are trained on geological information like seismic surveys, well logs, and satellite imagery. These models learn complex patterns within the data and generate realistic simulations of subsurface formations. With these simulations, the models can predict the likelihood of gas and oil reservoirs in specific geographic locations.
Also, geological datasets in gas & oil sectors are massive and require intensive computational processing. With GPU acceleration, companies can analyse these datasets rapidly, reducing analysis times from weeks to hours. GPUs designed to handle multiple tasks simultaneously through parallel processing, speeds up the complex analysis process.
With faster analysis of geological data, exploration teams can quickly identify potential resource-rich areas and make informed decisions regarding drilling operations and resource allocation. This accelerated decision-making process allows companies to capitalise on opportunities more efficiently. This scalability ensures that even as datasets grow in size and complexity, the analysis can still be performed swiftly and accurately without compromising on accuracy.
What Can Technolynx Offer You as a Software Company?
In the realm of energy and sustainability, TechnoLynx emerged as a pioneering force, offering tailored AI solutions designed to meet the distinctive demands of the energy industry. Our commitment lies in revolutionising the energy landscape through seamless integration of advanced technologies, driving efficiency, and sustainability to unprecedented heights.
At TechnoLynx, we boast unparalleled expertise in harnessing the power of AI across various domains, from Computer Vision to Generative AI, GPU acceleration to IoT edge computing, and from NLP to AR/VR/XR technologies. This multifaceted approach enables us to craft bespoke solutions that address the unique challenges faced by the energy sector, empowering organisations to optimise their operations and embrace sustainable energy AI solutions.
What sets us apart is our proven track record of delivering tangible results. Through our AI solutions, energy companies can experience a paradigm shift in performance, witnessing improved forecasting accuracy, enhanced operational efficiency, and substantial cost savings. By leveraging cutting-edge technologies, we enable our clients to make data-driven decisions, mitigate risks, and adapt swiftly to dynamic market conditions, thereby paving the way towards a more sustainable future.
To learn more about how TechnoLynx can help you revolutionise your energy management and drive sustainability, visit our contact form and start the conversation with our experts today.
Final Thoughts
In conclusion, the integration of artificial intelligence (AI) in energy management represents a transformative leap towards a more efficient and sustainable future. As we continue to harness the power of AI, the potential for a positive impact on energy and sustainability is immense. With advanced technologies, we not only improve the reliability and resilience of our energy infrastructure but also mitigate environmental impact and accelerate the transition towards a more sustainable energy ecosystem.
In partnership with TechnoLynx, the energy industry can unlock limitless possibilities, leveraging AI to meet not only current challenges but also pioneer transformative solutions that redefine the way we approach energy production and consumption. Together, let us embrace the power of AI to create a more sustainable and resilient energy ecosystem for all.
References:
-
Airbus.com. (2021). Airbus innovation for military aircraft inspection and maintenance [online].
-
California ISO. (2009). Renewable Resources and the California Electric Power Industry: System Operations, Wholesale Markets and Grid Planning California Independent System Operator Corporation.
-
Ebenus Design Solutions Private Limited (2023). Revolutionizing Maintenance with Artificial Intelligence: The power of Predictive Maintenance.
-
GE. (n.d.). Power Plant Software. Power Generation Solutions [online].
-
International Energy Agency. (2021). Net Zero by 2050 – Analysis [online].
-
Kara, T. and Şahin, A.D. (2023). Implications of Climate Change on Wind Energy Potential [online] 15(20), p.14822. doi:https://doi.org/10.3390/su152014822.
-
Mckinsey (2023). Economic potential of generative AI - McKinsey [online].
-
NERSC (n.d.). National Energy Research Scientific Computing Center [online].
-
NREL. (n.d.). Solar and Wind Forecasting [online].
-
OPG. (n.d.). Powering Ontario > Our power generation [online].
-
Sensoriafitness.com. (2014). Sensoria Artificial Intelligence Sportswear [online].
-
SolarEdge (n.d.). Solar Powering People’s Lives Around the USA [online].