AI Solutions for Battery Design & Maintenance Forecasting
Introduction
In this new era where energy demands continue to rise at faster rates, the integration of artificial intelligence (AI) is revolutionising the skyline of energy infrastructure. From reshaping battery design to enhancing the efficiency of smart grids and enabling predictive maintenance forecasting, AI technologies play a pivotal role in optimising energy systems.
This article explores how AI is driving innovation in battery technology, smart grid management, and maintenance forecasting, ultimately maximising the potential of our energy infrastructure.
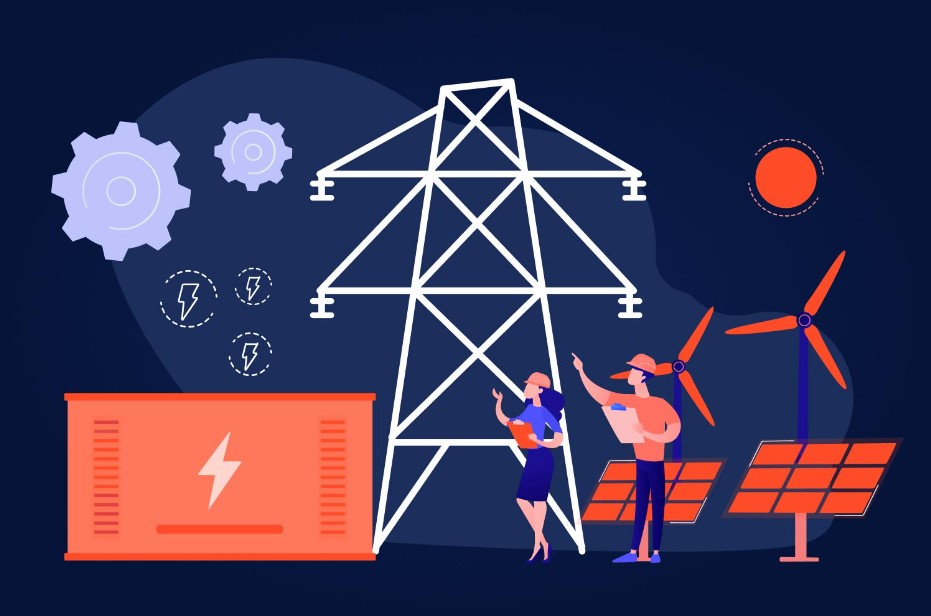
AI Solutions for Battery Design
Battery design requires the integration of Artificial Intelligence (AI) to address several critical challenges and enhance overall performance and efficiency. Traditional methods of battery development, which often span 5-7 years, are time-consuming, costly, and limited by the complexity of materials and electrochemical processes. AI can reduce development cycles by up to 50%, lowering them to just 2-3 years.
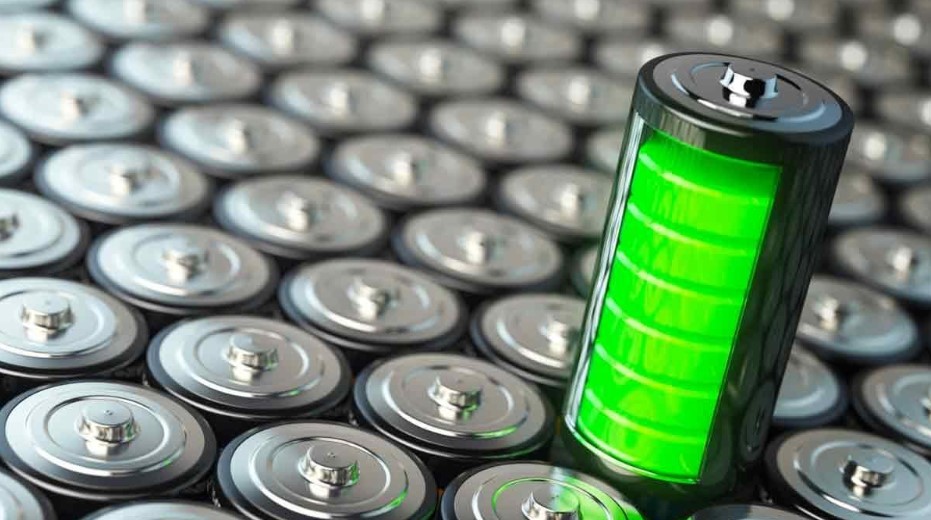
Optimising Battery Lifespan and Sustainability
Batteries are crucial for both renewable energy storage and electric vehicles (EVs), promoting green energy solutions. Traditionally, creating customised batteries has been challenging. However, AI is increasingly used to enhance the lifespan and sustainability of batteries.
To illustrate, scientists at Stanford are using generative AI to discover a novel lithium-sulphur battery material with 40% higher energy density and an approximately 25% shorter charging time compared with that of earlier electric vehicles. As a result, this marks an important step forward in electric vehicle technology.
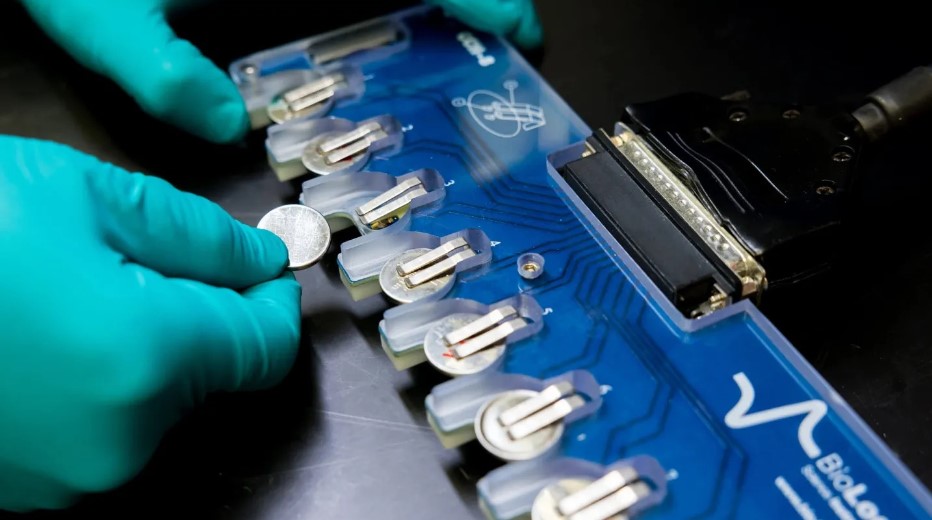
AI-Driven Simulations for Design Optimisation
AI simulations could improve a company’s understanding of how oil and gas reservoirs underground will act. By training on data from past drilling operations, AI can recommend the best methods for new wells, reducing accidents and improving worker safety.
Additionally, AI simulations predict the environmental impact of oil and gas activities, such as air and water quality or wildlife habitats. This helps companies minimise harm and meet regulatory requirements. By optimising supply chains, AI saves time and money while reducing the industry’s overall environmental footprint.
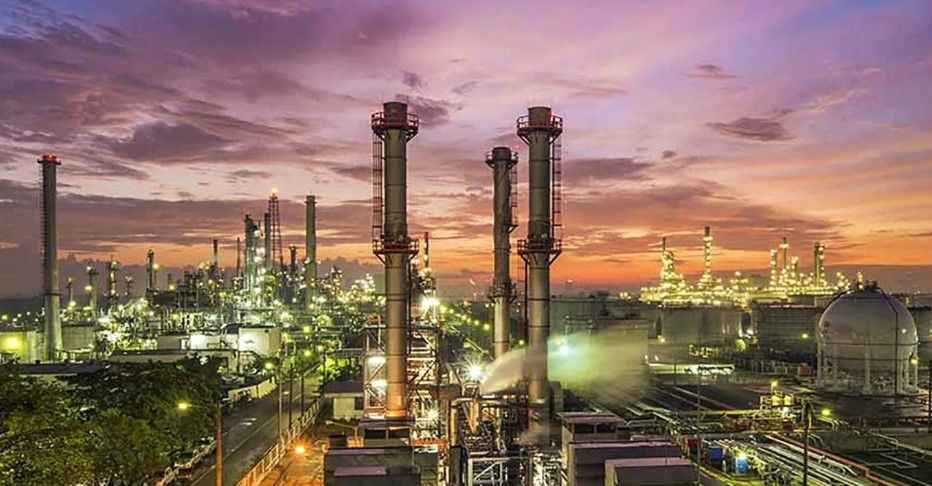
Generative AI for New Materials
The Generative AI in Energy Market, valued at USD 640.40 million in 2022, is projected to grow to USD 5,349.20 million by 2032. Generative AI acts as a creative partner for scientists, helping them discover new battery materials and structures.
By simulating material behaviour, scientists can identify materials with better energy storage, stability, and eco-friendliness. This accelerates the development of advanced battery designs and energy management systems.
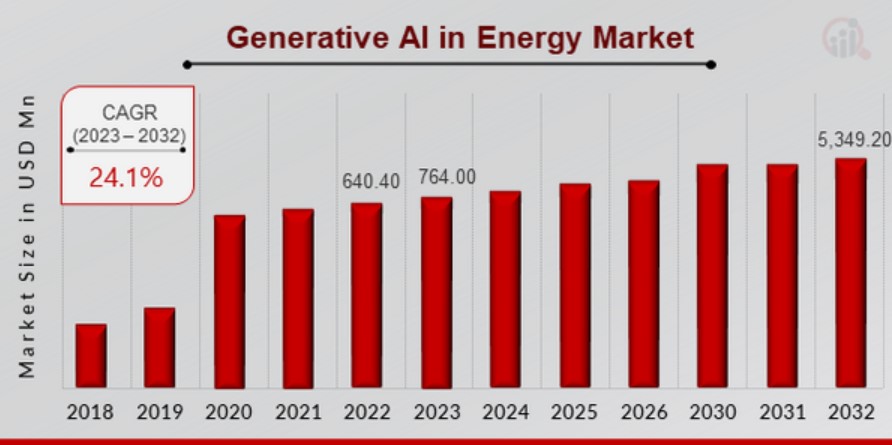
GPU Acceleration for Faster Development
GPU acceleration, like a turbo boost for cars, speeds up battery design. It allows computers to process complex calculations quickly, enabling researchers to test numerous battery designs rapidly. This accelerated testing process helps scientists develop new and improved batteries faster, contributing to a more sustainable energy future.
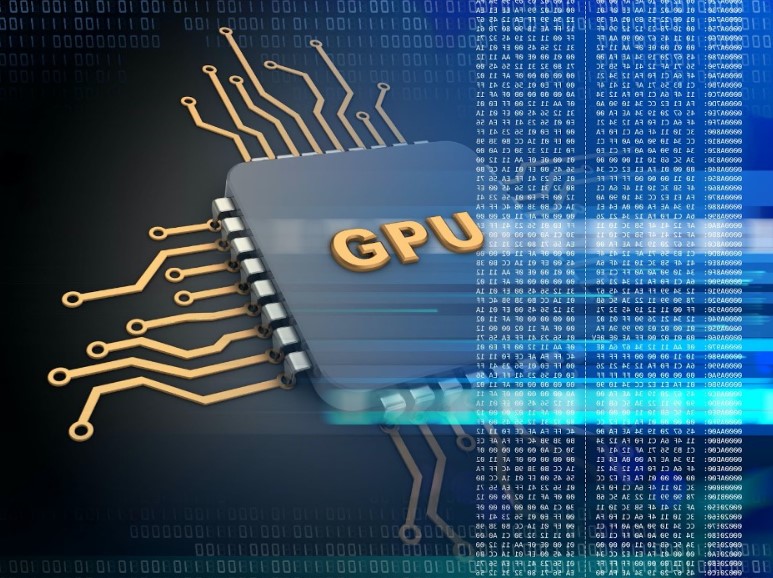
AI-powered Smart Grids for Energy Management
Smart grids need Artificial Intelligence (AI) to address inefficiencies in energy distribution, manage the complexities of integrating renewable energy sources, and reduce operational costs. AI enhances the accuracy of demand forecasting, advances energy flow, and predicts maintenance needs, thereby reducing energy losses by up to 30% and operational costs by 15-20%. This integration not only ensures a more reliable and efficient energy supply but also supports the transition to a sustainable energy future.
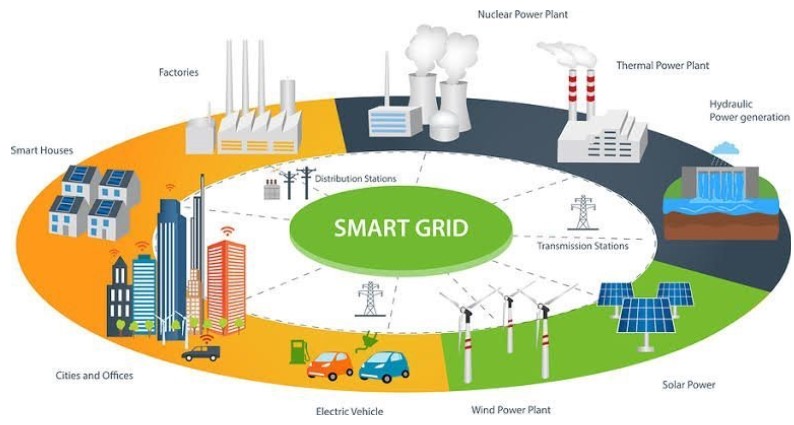
Real-Time Monitoring and Two-Way Communication
Smart grids installed at homes and businesses offer real-time monitoring of electricity usage for more accurate billing and management of energy consumption. Traditional power grids operate with a one-way flow of electricity from power plants to consumers, while smart grids integrate AI algorithms for two-way communication between power plants, substations, transformers, metres, and end-users. AI analyses data from sources like production rates, pipeline capacities, and storage levels to enhance energy distribution.
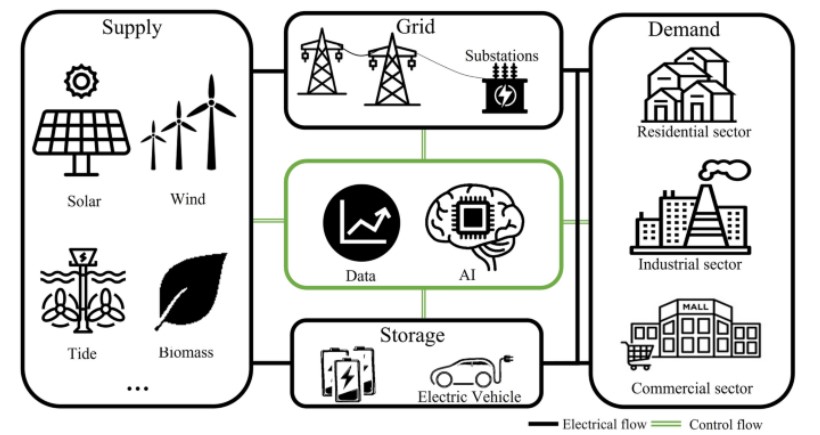
Optimising Energy Distribution
AI algorithms regulate the flow of natural gas and oil through pipelines, ensuring that energy resources are directed where they are needed most. This optimisation leads to more efficient energy distribution. AI also enables early detection of hazards, allowing operators to take preventive measures, avoid accidents, and ensure the smooth running of energy systems.
For example, the city of Chattanooga implemented smart grid AI algorithms, resulting in a 15% decrease in city-wide energy consumption and a 30% reduction in peak demand charges, demonstrating the efficiency of AI in energy management.
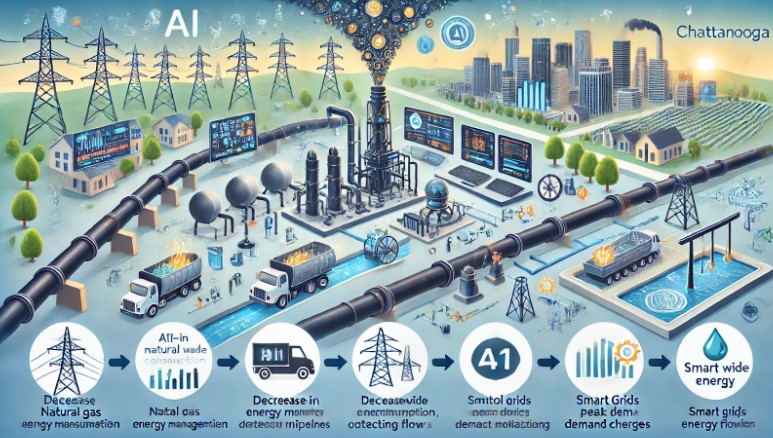
Weather Forecasting for Dynamic Load Management
Integrating weather forecasts into AI for smart grids allows energy providers to adjust electricity generation and distribution in real time. AI analyses vast amounts of weather data to predict electricity demand at different times.
For instance, on a hot day, AI can forecast increased air conditioner usage, requiring extra power. Conversely, during low-demand periods, such as at night, power generation can be scaled down to prevent energy waste.
This proactive approach helps prevent blackouts and reduces carbon emissions. In Denmark, integrating AI-driven weather forecasts into the national smart grid increased wind energy utilisation by 20% during peak production times, reducing reliance on fossil fuel-based power plants.
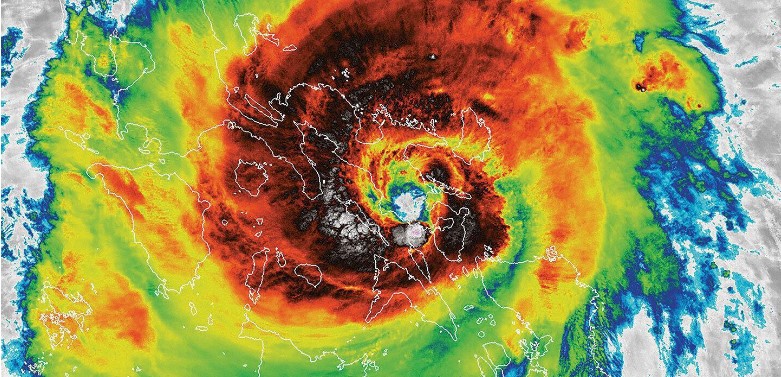
IoT for Energy Monitoring and Demand Analysis
IoT devices equipped with sensors collect real-time data on energy usage at various points within the grid. AI algorithms deployed at the edge analyse this data locally, identifying patterns, anomalies, and opportunities for energy consumption optimisation without the need for centralised processing.
This decentralised approach enables quicker response times, enhances grid resilience, and empowers consumers to make informed decisions about their energy usage, contributing to greater sustainability. ThingsBoard’s IoT energy management system uses AI to process real-time data from smart meters, surging energy consumption and reducing costs by up to 20%.
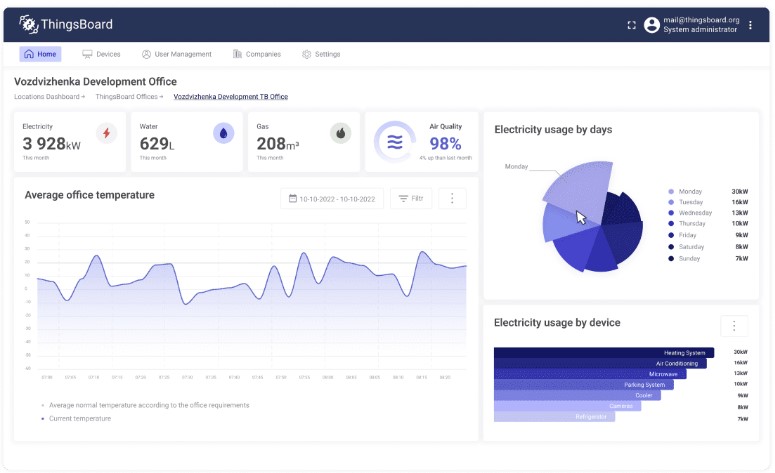
Natural Language Processing (NLP) for Unstructured Data Analysis
NLP algorithms in smart grids analyse unstructured data, such as customer feedback and market reports, to extract insights on demand-supply dynamics, trends, and consumer preferences. This enhances decision-making, pricing strategies, and infrastructure investments, boosting efficiency and sustainability.
The City-Zen project in Amsterdam renovated 10,000 buildings, saving 35,000 tonnes of CO2 annually. The Amsterdam ArenA’s smart grid uses AI and digital twins for energy efficiency, while the Flexpower system adjusts EV charging speeds based on real-time grid capacity.
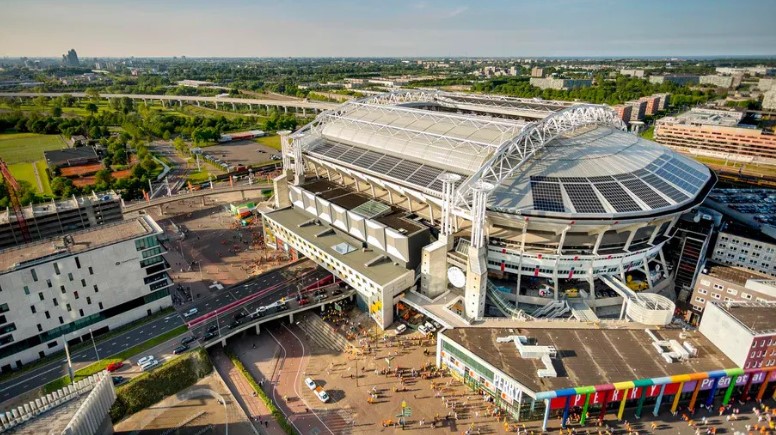
AI-Driven Maintenance Forecasting
Maintenance Forecasting is like predicting when your car might break down before it actually does. But instead of cars, we will discuss the big machines and equipment that keep our energy systems running smoothly below.
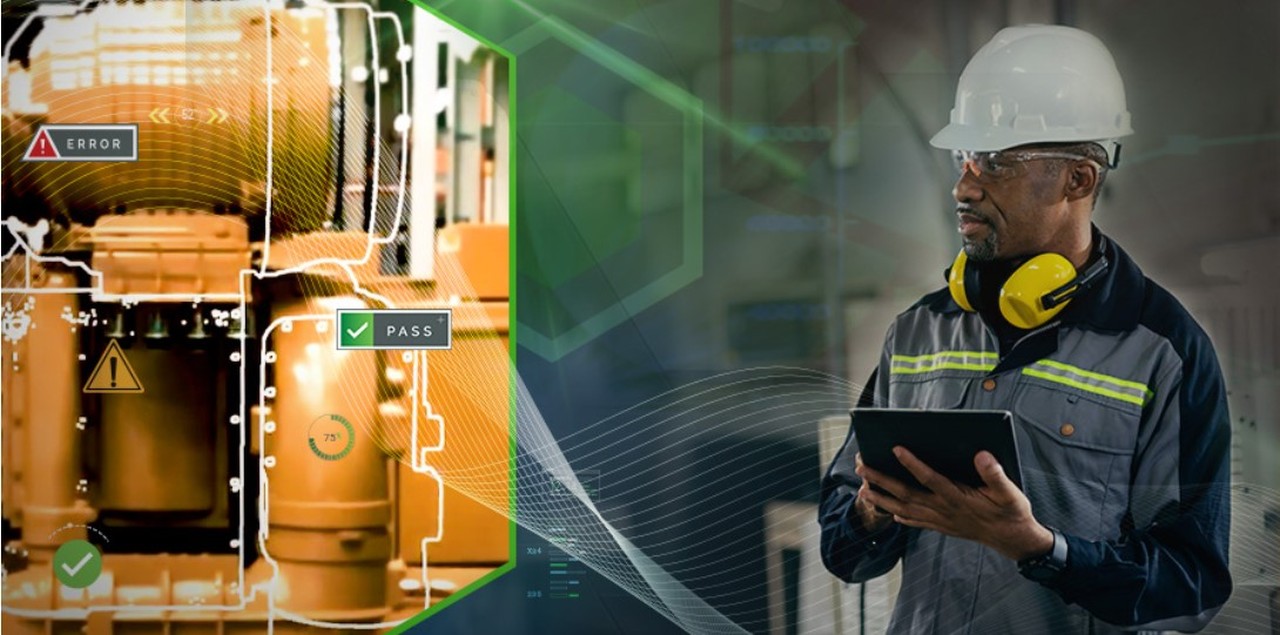
Predictive Maintenance for Energy Infrastructure
Maintenance forecasting is like predicting when your car might break down before it actually does, but applied to the large machines and equipment that keep our energy systems running smoothly. Instead of waiting for something to go wrong, AI takes a proactive role in analysing massive amounts of data from the energy infrastructure. AI algorithms can recognise abnormalities or patterns in the data, predicting potential equipment failures before they occur. This enables timely actions to prevent disruptions and enhance asset performance.
Historical records of machine performance, maintenance data, and environmental factors are invaluable for machine learning algorithms. These algorithms learn from past incidents to predict future failures with increasing accuracy over time.
For instance, the Norwegian power company Statkraft reduced maintenance costs by 20% and increased the operational lifespan of its hydroelectric turbines by five years using AI-fuelled predictive maintenance, contributing to more sustainable energy production.
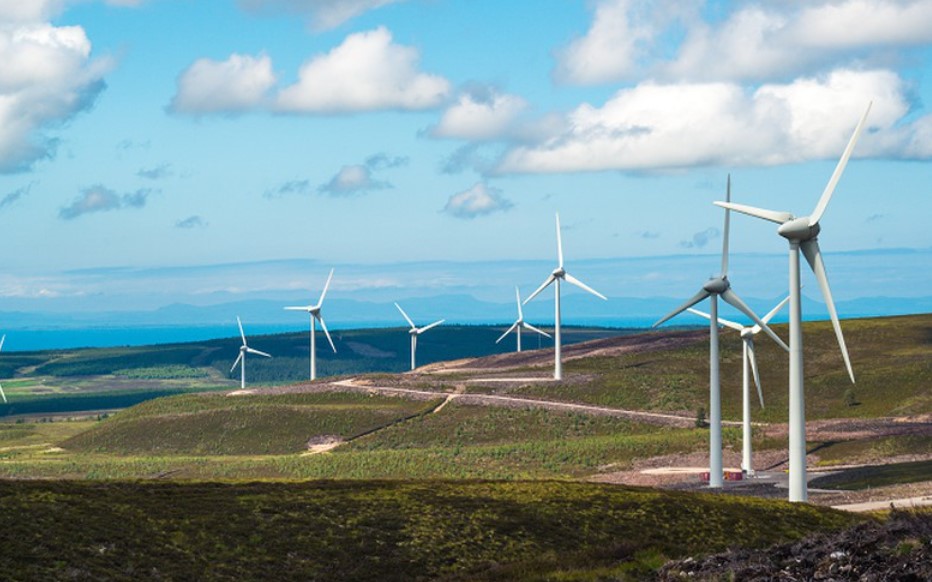
Visualising Maintenance Procedures with AR/VR/XR
Ever wish you had X-ray vision to see inside machines? With AR/VR/MR/XR technology, maintenance workers can wear headsets that show information about the machines they’re working on, like when they need maintenance or how to fix them. It’s like having a virtual instruction manual right in front of your eyes, making maintenance faster and easier. GE’s FieldCore division used AR/VR/XR technology, uplifting maintenance efficiency by 30% and cutting equipment downtime by 25%, saving $50 million annually.
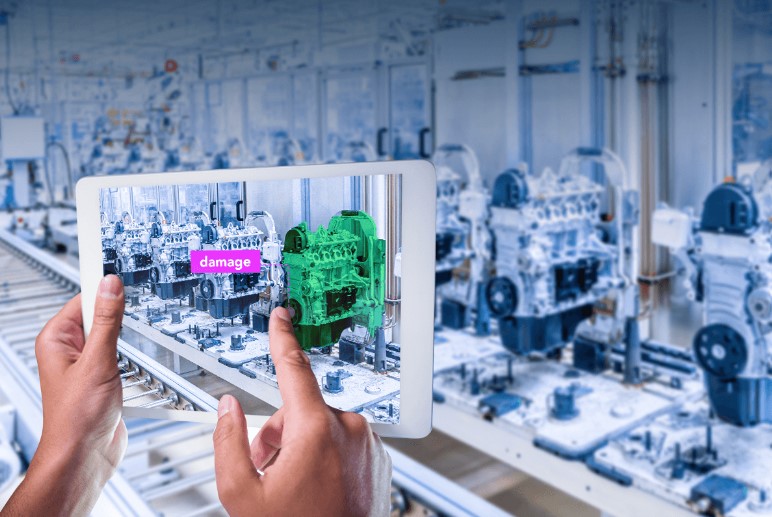
Benefits
-
Efficiency Improvement: AI-powered applications can help improve energy output, control, and consumption which in turn can enhance efficiencies and reduce wastage.
-
Cost Savings: Organisations will be able to predict maintenance requirements and enhance power consumption, leading to lower operational costs while maximising resource utilisation.
-
Sustainability: AI enables the effective grid integration of renewable energy sources, promoting sustainable energy and reducing CO2 emissions.
-
Reliability: The use of predictive maintenance and fault detection algorithms for energy infrastructure enhances the reliability of this system, delivering less downtime and better service reliability.
-
Innovation: AI fosters innovation in energy technology, leading to the development of advanced battery designs, smart grid solutions, and energy management systems.
Challenges
-
Data Quality and Availability: AI algorithms rely on the quality of big data, for instance, which may sometimes be difficult to acquire.
-
Complexity: The overall adoption of AI in the energy sector becomes a complicated process which demands knowledge in AI and energy engineering technology.
-
Regulation and Policy: There might be a gap between regulations and technological innovations which will result in difficulties of AI implementation in the energy sector.
-
Security Risks: Connected power grids are at risk of cyber-attacks, and AI-driven solutions may introduce new security challenges unless they are carefully implemented.
-
Ethical Considerations: AI algorithms may exhibit biases or unexpected effects introducing ethical challenges around privacy, fairness, and accountability in the decision-making process.
What We Can Offer as TechnoLynx
At TechnoLynx, our expertise lies in developing custom AI solutions tailored to diverse industries, including energy. Our team of AI specialists has extensive experience in designing and implementing innovative solutions to tackle complex challenges. We understand the importance of sustainability and efficiency in the energy sector, and we are committed to collaborating with industry partners to develop AI solutions that meet their specific needs.
Whether it’s optimising energy infrastructure, improving battery design, or enhancing energy management practices, TechnoLynx can work closely with clients to develop tailored AI solutions that drive positive outcomes. Our collaborative approach, combined with our deep understanding of AI technologies, ensures that our clients receive solutions that are both effective and sustainable. We are dedicated to supporting our clients in their journey towards achieving their sustainability goals and driving innovation in the energy sector.
Ready to revolutionise your energy infrastructure with advanced AI solutions? Contact TechnoLynx today to explore how our expertise can drive sustainability, efficiency, and innovation in your organisation. Let’s collaborate to unlock AI’s full potential in the energy sector and pave the way for a brighter, greener future. Reach out to us now to begin your journey towards optimised energy solutions.
Final Thoughts
AI technology, battery design, and energy management are vital building blocks on the path to a green energy ecosystem. They guide energy optimisation, battery charging, and energy management through intelligent systems like GPU acceleration, generative AI, IoT edge computing, and machine learning algorithms. From minimising the need for replacement and increasing lifespan to lowering operating costs and maintenance needs, AI has a measurable impact.
As we look to the future, AI’s role in energy sustainability will continue to evolve and expand. TechnoLynx remains committed to driving this evolution by developing tailored AI solutions that address the specific needs of our clients in the energy industry. Together, we can leverage the power of AI to create a more sustainable and efficient energy landscape for generations to come. Let’s embark on this journey towards a greener future, fuelled by innovation and powered by technology.
References:
-
FieldCore. (n.d.). Home FieldCore - A GE Company. Engineering & Field Service Solutions [Accessed 27 Mar. 2024].
-
GovTech. (2020). Chattanooga, Tenn., Picked for Smart Cities Tech Test [Accessed 27 Mar. 2024].
-
Lombardo, T., Duquesnoy, M., El-Bouysidy, H., Årén, F., Gallo-Bueno, A., Jørgensen, P.B., Bhowmik, A., Demortière, A., Ayerbe, E., Alcaide, F., Reynaud, M., Carrasco, J., Grimaud, A., Zhang, C., Vegge, T., Johansson, P. and Franco, A.A. (2021). Artificial Intelligence Applied to Battery Research: Hype or Reality? Chemical Reviews. doi:https://doi.org/10.1021/acs.chemrev.1c00108.
-
news.stanford.edu. (2024). Sitting idle boosts the performance of lithium metal batteries for next-generation EVs [Accessed 27 Mar. 2024].
-
Statkraft. (2024). Statkraft is planning record investments in Norwegian hydro and wind power [Accessed 27 Mar. 2024].