Introduction
Generative AI has shifted from being a futuristic concept to a reliable tool that is changing how businesses operate. Unlike traditional AI tools that analyse existing data, it can generate new content, ideas, and solutions by learning from various data. Its ability to create new content opens business possibilities worldwide.
For businesses looking for a competitive edge, Generative AI solutions can support creativity, simplify workflows, and offer more value to customers. In particular, businesses can speed up operations by automating tasks such as content creation, data analysis, and customer support.
We are seeing this happen in real-time. As customer expectations keep rising, many businesses are adopting Generative AI to create personalised experiences, dream up innovative products, and deliver more value than ever before.
In this article, we will take a closer look at Generative AI, its diverse applications, and its strategic value for businesses seeking sustainable growth and innovation.
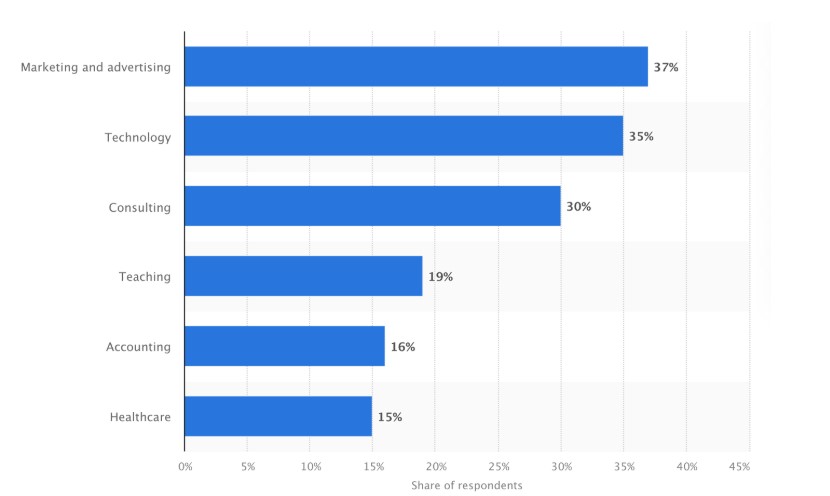
What is Generative AI?
Generative AI is a branch of artificial intelligence focused on creating new data by learning and replicating patterns from existing data. Generative AI models can produce different outputs, such as text, images, videos, music, code, and even 3D models, making it a versatile tool for innovation and creativity.
These advanced models rely on various types of data to create their outputs. Some generative AI models can even process multiple input types, from text and images to audio and code. Multimodal models are very popular at the moment, and they combine different inputs, like generating an image from a text prompt or improving it with a reference image. Similarly, videos can be created by merging text prompts with stock images.
How does this work? Under the hood of these models, they focus on first understanding existing data. A deep level of understanding is attained through cutting-edge technologies like neural networks and deep learning.
Neural networks are the basis of Generative AI tools. They enable models to learn intricate patterns and relationships within data. Meanwhile, deep learning builds on this by using multiple layers of neural networks to extract high-level features and generate context-aware outputs.
Similarly, various machine learning networks, such as Variational Autoencoders (VAEs) and Generative Adversarial Networks (GANs), play a crucial role in training Generative AI models. VAEs focus on learning data representations, while GANs use a dual-network approach - a generator and a discriminator to produce realistic outputs through an iterative process.
Read more: What is Generative AI? A Complete Overview
Types of Generative AI Models
Generative AI models are multifaceted and used for various applications across industries. Among the many types of models, the most common are text-based, image-based, video-based, and audio-based tools.
Let’s take a quick glance at these four types:
-
Text-Based: Generative AI is widely used in text generation and natural language processing (NLP) applications. These models can be used to create stories, articles, poems, scripts, code snippets, and chatbots for language translations, text summarising, and human-like interactions.
-
Image-Based: Image generation uses techniques like GAN and diffusion models to create images for professionals in art and design. These models can also support features like editing existing images, enhancing their quality, and transforming them into different themes and styles.
-
Video-Based: Video generation can be thought of as an upgraded version of image models. These models can create videos from still images. It is used to generate special effects and 3D animation for movies. Like image-based models, video-based models can also be used to edit existing videos.
-
Audio-Based: Music-based or audio-based Generative AI focuses on creating and manipulating audio information. These models can be used to compose music pieces and generate sound effects and background soundtracks.
How Generative AI Drives Business Growth
Generative AI, with its creative capabilities, is redefining the way businesses operate. It offers innovative solutions that help drive growth, improve efficiency, and enhance customer engagement. Let’s walk through some examples.
Improving Efficiency and Innovation
Businesses are using generative AI in the form of large language models (LLMs) to automate tasks that used to consume a lot of time. LLMs can take over mundane tasks like data entry, report generation, and customer support enquiries. They can also analyse vast datasets to identify trends, predict outcomes, and inform crucial business decisions. Adopting LLMs gives employees more time to focus on the strategic side of business operations.
However, this isn’t just with respect to monotonous tasks. For example, in industries like gaming and media, AI tools can be used to generate realistic characters, virtual environments, or even entire storylines. Using generative AI in this line of work can substantially reduce video game production time and development costs. Creative professionals like artists and designers can use generative AI tools to explore new creative ideas, experiment with different styles, and efficiently bring their imagination to life.
Customer Service Transformation
Text-based and conversational AI can replace the need for extensive manpower in customer service by handling interactions more efficiently. AI chatbots and virtual assistants can provide 24/7 support, answer frequently asked questions, and resolve common issues instantly. With this, businesses can reduce waiting time and improve customer satisfaction.
A great example of this is Amazon’s AI-powered chatbot, Rufus. You might have even used Rufus’ help when you were shopping. By putting together its generative AI capabilities with Amazon’s extensive catalogue and web knowledge, Rufus aims to simplify the shopping experience.
Rufus is also a good case study for AI assistants that focus on hyperpersonalization. It figures out what the customer is searching for and provides relevant suggestions in real-time. It fits recommendations, comparisons, and insights with individual customer needs, creating a seamless and engaging shopping experience.
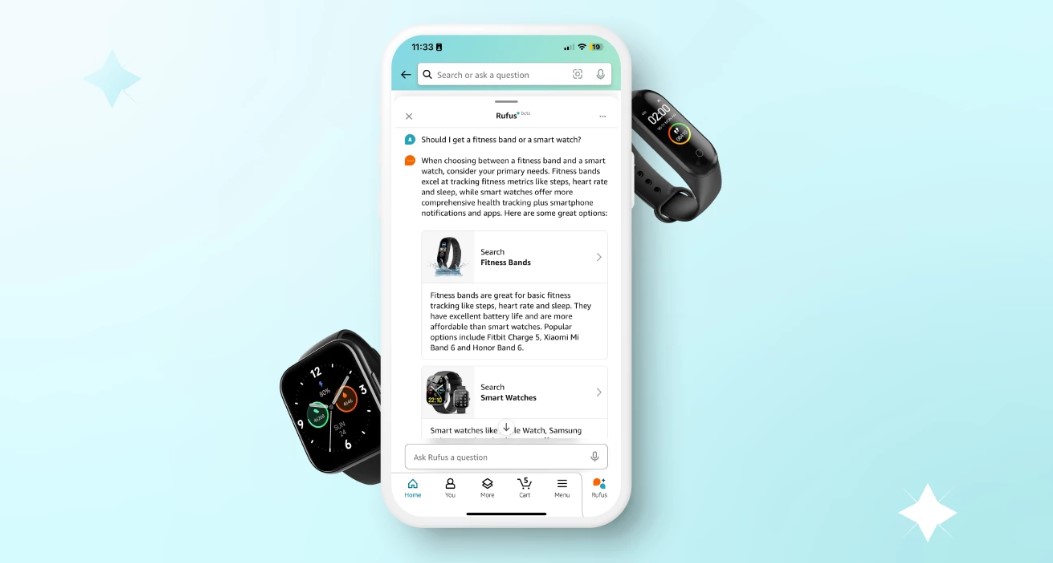
Synthetic Data for Training and Scalability
The development and training of AI models require huge volumes of real-world data. However, acquiring high-quality data can be costly and often challenging due to various factors like data scarcity, privacy, and bias.
Interestingly, synthetic data, artificially generated datasets that mimic real-world data characteristics and patterns, can overcome these limitations. Generative AI tools can be used to create synthetic datasets that are customisable and scalable for specific applications.
Let’s say you are working on a self-driving car. Synthetic image data can be generated to simulate diverse driving scenarios, including road conditions, weather patterns, lighting variations, and potential obstructions. The synthetic data can be used to train a computer vision model for self-driving cars.
Synthetic data can also help stimulate imaginative or rare events that are difficult or impossible to capture. As a matter of fact, models trained on extensive synthetic datasets that account for a wide range of scenarios help improve decision-making and achieve better outcomes.
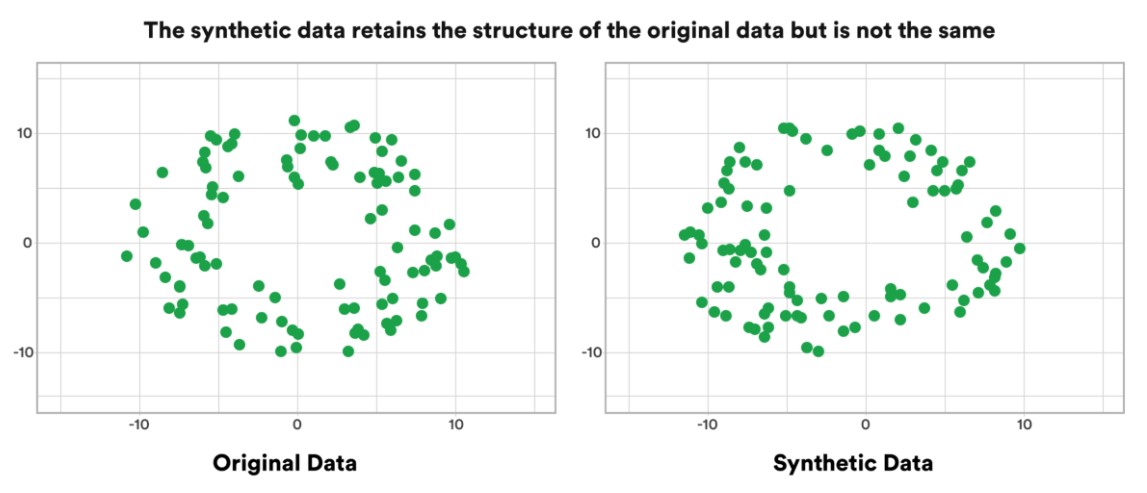
Read more: Generative AI vs. Traditional Machine Learning
Generative AI Applications Across Industries
Next, let’s discuss some key areas like healthcare and e-commerce where Generative AI is making an impact.
Entertainment and Media
We’ve touched upon how generative AI tools can be used creatively in the entertainment industry, from filmmaking to music creation. Diving into the details, Generative AI models can increase the pace of the production process by generating different types of content, such as images, music, videos, and animations. Filmmakers and production teams, for instance, can use generative AI to create high-quality visual effects, design lifelike characters, and automate tedious production tasks like script writing and storyboarding.
Similarly, in the music streaming industry, platforms like Spotify are using Generative AI to improve the user experience. Spotify’s AI DJ feature analyses a user’s listening history, such as favourite genres, artists, and tracks. Based on this data, it creates personalised playlists that suit the listener’s preferences. As users continue to interact with the platform, the Generative AI model learns and refines its recommendations for a better experience.
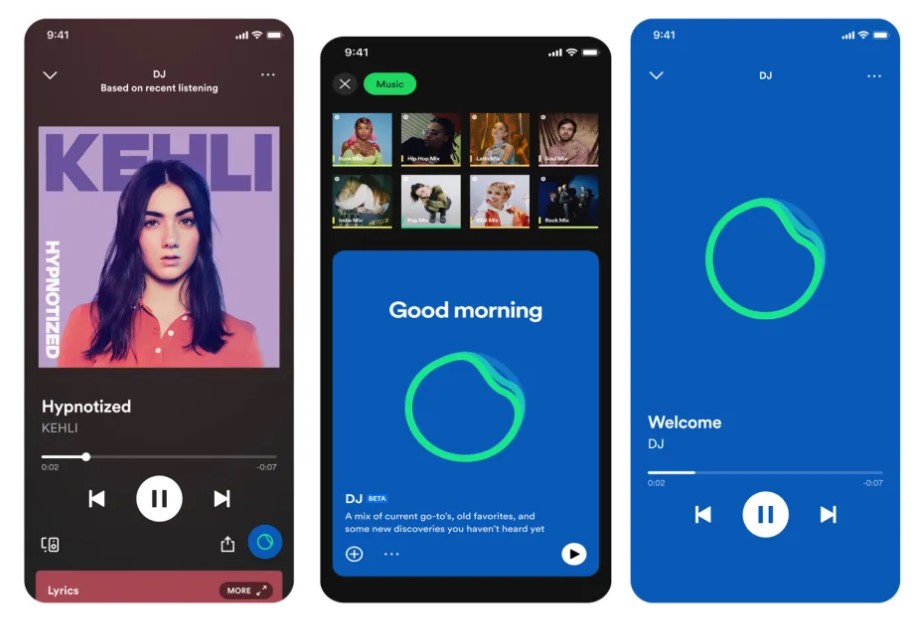
Read more: How Artificial Intelligence Transforms Social Media Today
Healthcare and R&D
Generative AI is increasingly being used in healthcare, especially for drug discovery. By analysing large amounts of medical data, Generative AI models can help find potential drug candidates and predict how well they will work.
What’s the process behind this? Generative AI supports drug discovery by improving molecular properties and simulating biochemical interactions. It analyses large datasets of molecules and biological information to find patterns. These patterns help predict how molecules will behave in different conditions.
This application of Generative AI speeds up research and helps to develop life-saving medications faster. With advanced model architectures and powerful GPUs, healthcare professionals can quickly test complex biological processes that can lead to faster breakthroughs and better health outcomes.
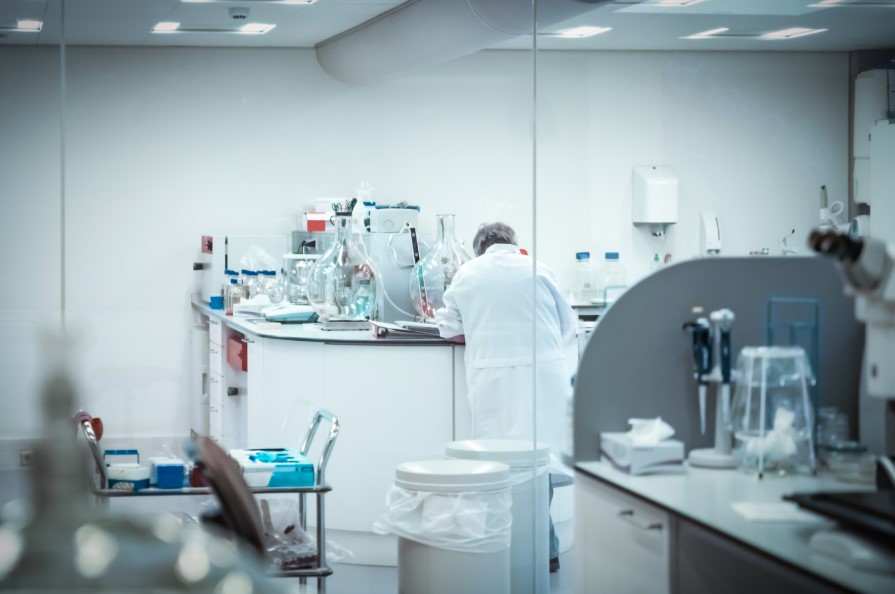
Marketing and E-Commerce
When integrated with Natural Language Processing (NLP), Generative AI models can generate text-based responses for specific applications. We’ve seen this in LLM-based applications like ChatGPT. Using such integrations, businesses can send personalised messages to every customer, such as recommending products or offering customised support. Businesses can even use these tools to create dynamic email content that aligns with individual customers, enhancing satisfaction and brand loyalty.
For example, ASOS (a retail brand) uses Generative AI to improve the shopping experience with its AI Stylist feature. This tool looks at customer’s style preferences to suggest outfits that suit them. Acting like a virtual assistant, the AI Stylist offers ideas based on the customer’s liking. Also, ASOS uses Generative AI to recommend billions of products to each customer every day. With Generative AI, shopping is more engaging and suited to individual needs.
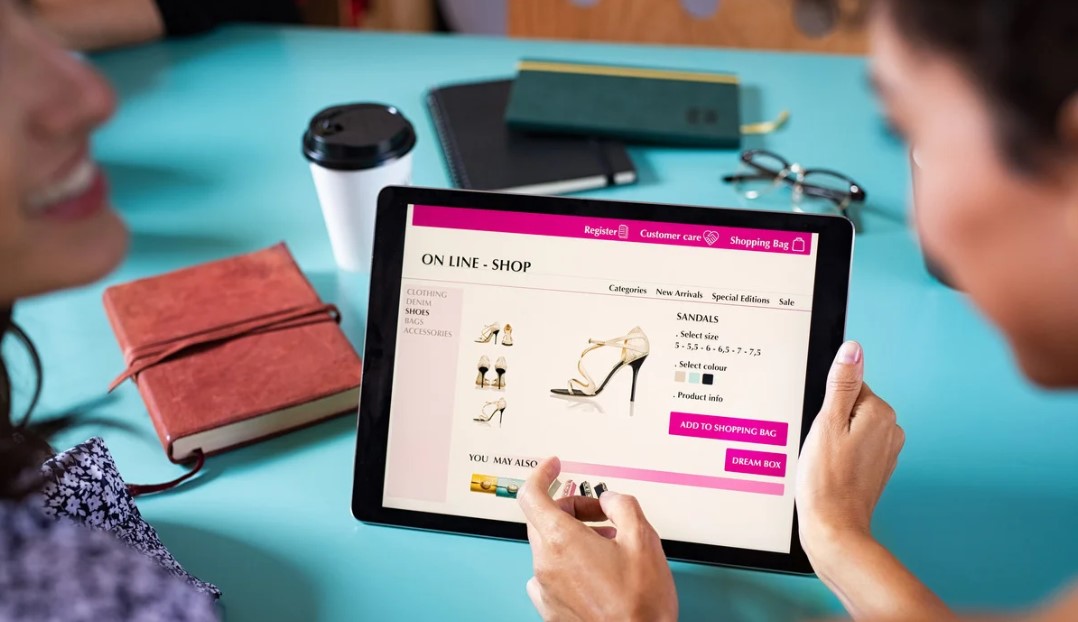
Generative AI tools can also be integrated with AR (augmented reality), VR (virtual reality), MR (mixed reality), and XR (extended reality) solutions to offer immersive and personalised experiences. In retail, this could mean helping customers virtually try on clothes, see how furniture would look in their homes, or explore customised product options in a realistic way.
Read more: Smart Marketing, Smarter Solutions: AI-Marketing & Use Cases
Challenges and Limitations of Generative AI
While Generative AI has the potential to make a big impact, it also comes with challenges and limitations. As AI technology develops, it’s important to address these concerns while exploring new possibilities and innovations.
Here are some key challenges that need to be tackled with respect to Generative AI:
-
Ethical Considerations: Generative AI can create highly realistic but fabricated images, videos, and audio files. But, these files can be misused to spread false information, manipulate public opinion, and damage reputations. Clear regulations and strong safeguards can prevent misuse while making it possible for Generative AI to be used responsibly and positively.
-
Dependence on High-Quality Data: In general, AI models depend on large amounts of high-quality training data. However, techniques like model optimisation and transfer learning are helping to reduce these demands and make AI technology more accessible to a wider audience.
-
Regulatory and Intellectual Property Issues: The legal ownership of AI-generated content is still unclear. Questions about copyright ownership, potential infringement of existing works, and the ethical use of copyrighted material in training data remain unresolved. However, these issues are being actively addressed, making it important to stay informed and aware of developments in this space.
Dealing with the challenges of generative AI often calls for the expertise of a tech professional to ensure everything runs smoothly. That’s where Technolynx comes in, providing the support and solutions businesses need to make the most of generative AI.
What TechnoLynx Can Offer
At TechnoLynx, we create custom Generative AI models tailored to meet the unique needs of your industry. Our focus is on building reliable, scalable AI systems that easily integrate into your existing workflows. Whether it’s chatbots, content creation, or improving customer interactions, our solutions are designed to be user-friendly and effective.
Beyond Generative AI, we also specialise in areas like deep learning, computer vision, and GPU acceleration, offering business-specific solutions to help you stay ahead. To ensure our solutions perform at their best, we prioritise optimising for speed and quality so your systems run efficiently and deliver excellent results.
Our mission is to help you unlock the full potential of AI, boosting creativity, improving efficiency, and giving your business a competitive edge. Let’s work together to scale your business with innovative AI solutions. Reach out to us today!
Future Outlook: Generative AI and Business Growth
Generative AI is still in its early stages, but many businesses globally are adopting it. These tech advancements will continue to change industries and shift how businesses compete. As technology improves, it will help companies innovate and stay ahead in the business.
When integrated with emerging technology trends like IoT (Internet of Things), edge computing, augmented reality (AR), and virtual reality (VR), Generative AI will likely play a vital role in creating immersive experiences.
We are already seeing large language models (LLMs) becoming more advanced in understanding human language. These steps forward are leading to more human-like interactions and improved decision-making capabilities.
Conclusion
In recent years, Generative AI has become a reliable tool for accelerating business growth, enhancing creativity, and driving innovation. Businesses can automate tasks, improve customer interactions, and overcome critical challenges using Generative AI tools. Generative AI models are expected to grow quickly in the near future. For businesses, it’s important to adopt these solutions and take advantage of their potential for sustainable growth.
At TechnoLynx, we specialise in helping businesses make the most of generative AI. From integrating the right tools to offering clear guidance, our solutions are customised to help you achieve your unique goals. We focus on providing practical and impactful AI strategies tailored to your business needs. Contact us now to learn more!
Sources for the images:
-
Amazon, 2023. Rufus AI Shopping Assistant Launch in India. About Amazon
-
Freepik, n.d. Face recognition and personal identification collage. Freepik
-
Leight, E. (2023) ‘Spotify’s New ‘DJ’ Pairs AI-Powered Commentary With Song Picks’, Billboard, 22 February.
-
o1559kip (n.d.) ‘Scientists in the laboratory are developing vaccines against coronavirus’, Envato Elements
-
Rido81 (n.d.) ‘Friends shopping shoes online’, Envato Elements
-
Rinf.tech, 2023. How Synthetic Data Solves Real-World Data Challenges (From Scarcity to Security). Rinf.tech
-
Thormundsson, B. (2024) ‘Generative AI adoption rate at work in the United States 2023, by industry’, Statista, 10 May.
References
-
Amazon, 2023. Rufus AI Shopping Assistant Launch in India. Amazon
-
Alvarado, J. (2023) ‘ASOS Case Study: AI Technology Triples Revenue Growth’, RetailBoss, 10 May.
-
Ren, F., Aliper, A., Chen, J., et al. (2025) ‘A small-molecule TNIK inhibitor targets fibrosis in preclinical and clinical models’, Nature Biotechnology, 43(1), pp. 63–75. Available at https://doi.org/10.1038/s41587-024-02143-0
-
Spotify (2023) ‘Spotify debuts a new AI DJ, right in your pocket’, Spotify Newsroom, 22 February.