Introduction: AI’s Role in Healthcare and Medicine
The healthcare field is definitely one of the most respected worldwide, which is why the healthcare industry is so big! Physicians and healthcare professionals have been respected since ancient times. How ancient? Well, the world-famous Hippocratic Oath dates back to the 4th century BC. ‘I will use therapy which will benefit my patients according to my greatest ability and judgment, and I will do no harm or injustice to them’, says the Oath (Greek Medicine, no date).
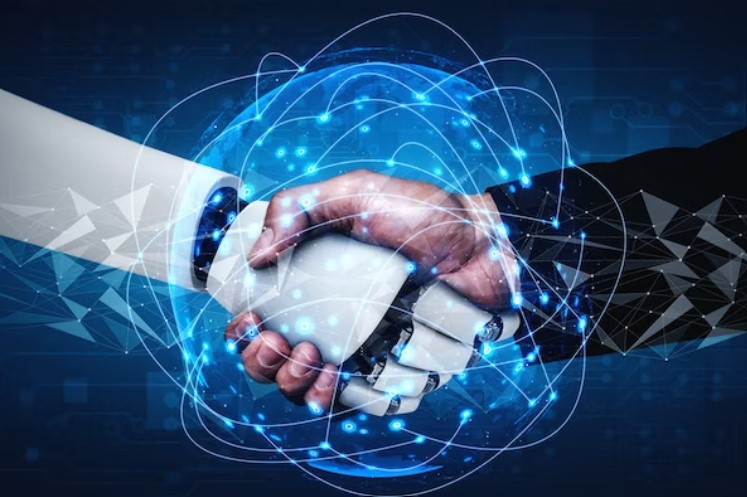
We have seen how medicine has changed over the years. Our society has evolved from digesting roots and trepanning for therapeutic purposes to visualising our internals with cutting-edge technology that produces extremely crisp images. What is the next step? The integration of AI into our arsenal for medical decisions, of course! Keep scrolling to find out more.
With Proper Training Comes Great Results
The first thing most people think about when they hear the word AI is something high-tech, and you know what? They would be right! AI is the theory and development of computer systems capable of performing tasks that normally require human intelligence, such as visual perception, speech recognition, decision-making, and language translation. ‘And how is that achieved?’ we hear you ask. The answer is hidden in a method you have probably already heard of that teaches computers to process data in a way inspired by the human brain: Deep Learning (DL). Before we dive deeper, we need to get a little technical, possibly geeky. We know you came here for the main course, but, trust us, you will find the appetiser very interesting.
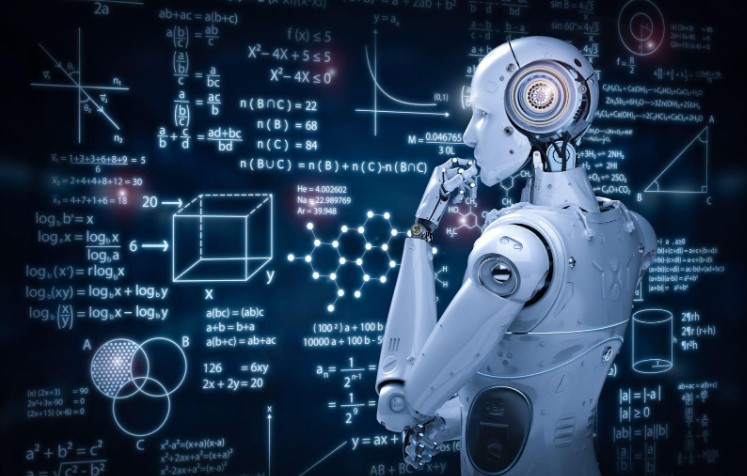
“I Will Make a ‘Man’ Out of You!”
Each AI algorithm needs proper training to perform its wonders. Optimally, this is achieved by creating an algorithm that can be trained on hundreds of thousands, if not millions, of data. To do that, we first must ensure that the data we feed the algorithm properly. This means that the data must be collected from various sources, such as databases, and that the data are ‘clean’. To do that, we need to check that there are no missing values or inconsistencies, that the classes are meaningful, and the labels are correct. The data are then transformed with techniques that normalise them, reduce their dimensions, or augment the data while ensuring no information is lost or wrongfully duplicated. Finally, the data are divided into train and test sets, and adjustments are made to ensure maximum accuracy with the minimum number of resources used. So far so good? Nice! Let’s move on.
Going beyond human!
We might want to make the most efficient and infallible AI algorithm for medical imaging. But what happens when the data are simply not enough? Well, it is not called AI for no reason! One of the best features of AI is Data Augmentation (DA). Generative AI models can alter existing data to generate new ones, but that is not all! One of the most powerful features of generative AI is Synthetic Image Generation (SIG). The difference between DA and SIG is that, instead of altering existing medical images, SIG can create synthetic medical images using the limited resources it has been provided with. Bless creativity!
The Incorporation of AI in Modern Medical Tech
Deep Learning (DL) and Computer Vision (CV), a GPU-accelerated pipeline of AI, have been used extensively in medical facilities by integrating them into medical Decision Support Systems (DSS). Such systems are embossed in most modern medical tech gear with the sole purpose of helping physicians and medical staff make the right decision at the right time. AI is defined by its ability to learn from large datasets and make decisions. Its computational power on numbers could be analogous to what we humans call ‘experience’. AI algorithms can run through millions of patient records and make decisions about their health status simply by looking at the input data. Although the results can be stunning, there is a way to push this beyond limits, called ‘Edge Computing’. Medical facilities have their servers and databases for the localised processing of data. Having them up to date hardware-wise allows the processing power to be maximised while minimising the time consumption. In this way, we optimise the performance of the AI algorithm with instantaneous results!
I See it All, I Know it All!
Medical imaging is one of the fanciest applications of CV. At least once in your life, you surely have had to have an X-ray, right? If you recall, the doctor would place your X-ray in a view box and carefully try to identify possible abnormalities. That’s ok, for sure, but is it even allowed in the digital age? Modern-age doctors have been shown to prefer DSS algorithms over the standard procedure that has been followed for many years. The reason is very simple: automation. CV can be trained to perform image analysis to automatically detect these abnormalities. Notice that we said ‘detect’. Not only can it identify which image has an abnormality, but it can also pinpoint with extreme precision where the abnormality is located! In one phrase: Computer-Aided Diagnosis (CAD). With a well-trained DSS pipeline, CV‘s benefits are multiple: Time-saving? Check! More accurate? Double check! The best part is that such algorithms can be set to be trained by learning from their mistakes. A doctor would not risk a machine-caused error. By interacting with the algorithm, it can be taught to recognise and never repeat the same mistake in real time!
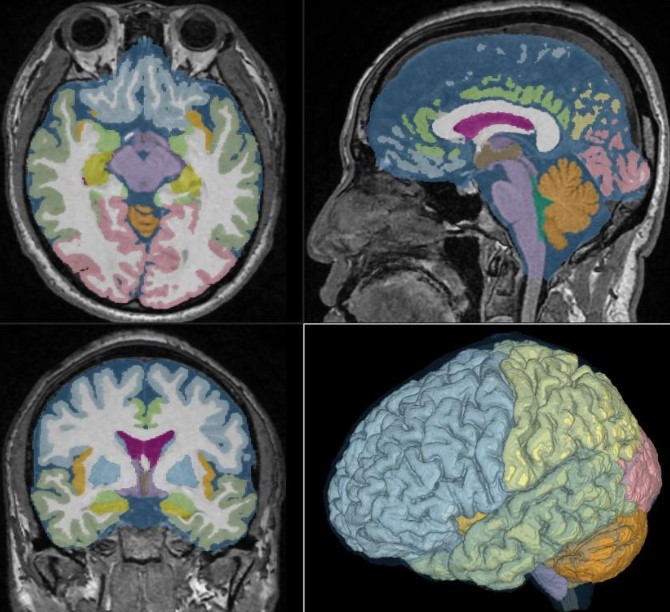
My Game, my Rules… My Risks?
Although we have shown what practical applications AI can have in medical imaging and CAD, nothing comes without a cost. As mentioned, great training comes with great results, but let us not forget that ‘with great power comes great responsibility’. Such a powerful tool as AI has its risks that must be addressed. And no, we will not talk about AI taking over and leaving us unemployed. The thing is that even though AI is so smart, it can sometimes be challenging to train. The challenges lie mostly in the lack of data, which, surely enough, can be countered with DA and SIG, as we already mentioned. However, the biggest threat to AI is something that you might or might not expect. If your guess was ‘humans’, you would be right. Human error remains a threat to the proper training and use of AI. Think of AI as a recipe for food. Despite executing it word by word, the meal will be a disaster if you add a ton of salt and pepper! Now take this and multiply it by a zillion times. After all, we are talking about human lives. Automation is good and all, but if a tiny issue can mess up one patient’s results, imagine what it would do to an entire medical facility with thousands of them.
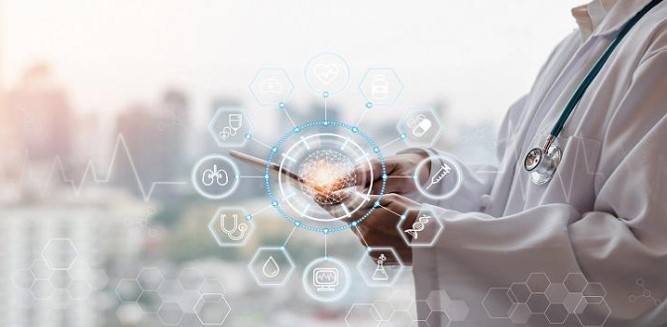
Summing Up
AI is a powerful ally in the field of medicine and healthcare. It can perform classification and segmentation tasks on medical images and screening, generate artificial images, and even correct its errors. In a nutshell, AI can undoubtedly almost run the diagnostics of an entire medical imaging facility on its own. By providing enough training information and having the necessary resources, there is no task AI cannot do.
What We Offer
At TechnoLynx, we specialise in delivering custom, innovative tech solutions tailored to any challenge because we understand the benefits of integrating AI into medical applications and healthcare institutions. Our expertise covers improving AI capabilities, ensuring safety in human-machine interactions, managing and analysing extensive data sets, and addressing ethical considerations.
We offer precise software solutions designed to empower AI-driven algorithms in various industries. Our commitment to innovation drives us to adapt to the ever-evolving AI landscape. We provide cutting-edge solutions that increase efficiency, accuracy, and productivity. Feel free to contact us. We will be more than happy to answer any questions!
List of references
- ‘Aging-related volume changes in the brain and cerebrospinal fluid using AI-automated segmentation - AI Blog - ESR | European Society of Radiology %’ (no date) ESR | European Society of Radiology (Accessed: 25 January 2024).
- Building smarter machines (2019) P.C. Rossin College of Engineering & Applied Science (Accessed: 28 January 2024).
- Evaluation of AI for medical imaging: A key requirement for clinical translation (2022) (Accessed: 28 January 2024).
- Greek Medicine (no date). U.S. National Library of Medicine (Accessed: 21 January 2024).
- How AI Helps Physicians Improve Telehealth Patient Care in Real-Time, telemedicine.arizona.edu (no date) (Accessed: 25 January 2024).